A Multiperspective View on AI Startups

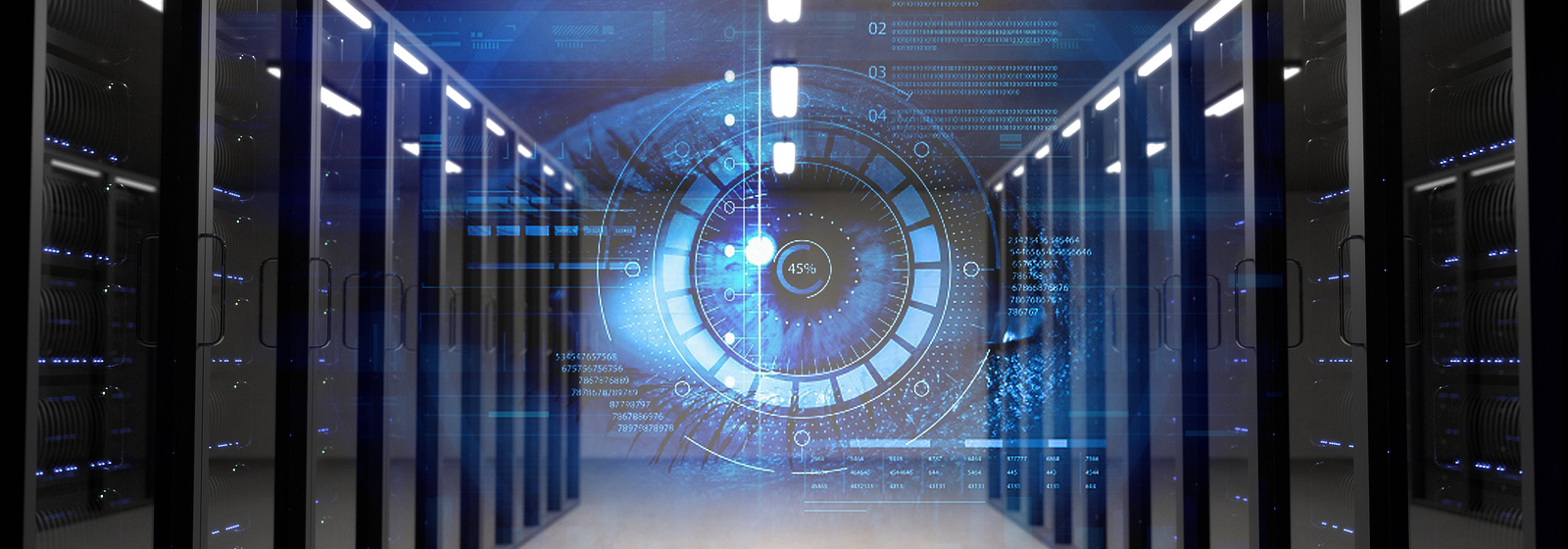
A Multiperspective View on AI Startups
Artificial Intelligence (AI) is the type of phenomena that is partially mystified by its novelty and sometimes reduced only to aspects that immediately fit existing cognitive frameworks. Prophetically announced as an inevitable part of the future world, AI is rarely considered by confronting more than just a few perspectives positioned in a broader social, economic, and technical context.
When assessing opportunities opened by new technologies, a reasonable approach is to rely on well established frameworks built on years and years of research and practice. However, routinely applying such a structured way of thinking only to several aspects, which are either obvious or directly resemble known concepts, represents a risks of missing potentially significant opportunities hidden in some essential technical nuances or in higher-level values that technology could bring to societies and industries. Those values may lie far from the technology itself and thereby stay invisible when focusing on the equally important lower levels.
For said reason, a multiperspective view which simultaneously encompasses various dimensions on different contextual levels seems as a necessary approach for identifying a short-term direction towards maximizing potential values of the technology — AI in this particular case. Yet, the purpose of this post is not to lay a bet on the version of the future that AI startups could optimally conform, but to present a possibly incomplete and to some extent speculative multiperspective view that can serve as a basis for deeper considerations and that demonstrates one possible way of thinking about the topic.
Closing the Gaps
A starting point that can open some interesting questions for constructing a multiperspective view is a boldly articulated hypothesis that the era of startups has reached its end because none of the predominant forces that constitute the new wave of technological changes are accessible to startup disruption. Besides AI, the referred promising fields are augmented/virtual reality, drones, autonomous vehicles, the Internet of Things, and blockchain technology. This vague and simplified statement about the accessibility to disruption requires a deeper consideration and it will be unwound in the following sections.
However, the notion of the upcoming end of the startup era undoubtedly seems to be provoked by the current market trends. The dominance of big tech companies is becoming more evident each day, especially since Apple has recently become the first and then Amazon the second publicly traded American company with a market capitalization of more than $1 trillion. The combined valuation of “the big five” tech companies — Apple, Alphabet, Microsoft, Facebook, and Amazon — reaches over $3.3 trillion which roughly makes a half of the gains achieved by the Standard & Poor’s 500-stock index. They have taken strong complementary positions in the market propelling the broader economy with their enormous growth.
From that perspective, it seems like big tech companies are overtaking all the disruption and closing market gaps for entrants. This may be partially explained by the accumulated capital, talents, resources (most valuable of which are data in case of AI), and the socio-political influence. On the other side, the consolidation trends are not exclusive to the tech sector. Two decades ago, the top five American banks controlled a little more than one fifth of the financial market, but today the top five banks hold almost half of all the assets.
Such a dramatic consolidation also happened in the airline and telecommunication industries during a similar timespan. According to a 2017 research by Jan De Loecker of Princeton and Jan Eeckhout of University College London, the difference between manufacturing costs and market prices are at its highest level since 1950 for American companies, indicating the increasing power that companies possess in their markets. The hypothesis that the pendulum swings farther from startups towards bigger companies on the global level is also supported by the assessment that seed funding slowed down in 2017.
In this light, it may seem idiosyncratic that the steady rise of funding in AI startups grew exponentially during the last year. Led by $620 million investment in Chinese facial recognition developer SenseTime Group and $153 million investment in American robotic automation company UiPath, such trends continued in 2018. Without considering global phenomena like the emerging dominance of big companies and recently weakened seed funding, decisive optimism in AI entrants may seem obvious, but in the broader context it exhibits anomalic characteristics. Despite many impediments that are idiomatic to AI startups, while being inconsequential for companies with enough capital, talents, data, and influence, investors apparently still believe that startups can turn impediments into opportunities.
Although these confronting perspectives based on global trends and financial indicators do not convey operative information that could help startups make strategic decisions, they provoke important questions about underlying causes of these phenomena. Why do startups have lower chances for accessing disruption in the promising fields and how should they choose the optimal positions to leverage the specific market situations and technical possibilities? Putting some effort in answering these questions, at least partially, motivates a step beyond the obvious answers and helps constructing a more comprehensive, multiperspective view.
Talent Shortage
It is impossible to read news about AI without encountering several repeating topics that occur both in the mainstream media and on specialized tech portals. The scarcity of talent in AI is apparently one of such topics, as it strongly affects not only startups, but companies of all sizes striving to seize the potential of AI. The phenomenon is not too peculiar, due to the amount of knowledge being accumulated in the related research fields at an enormous rate during the last several decades. Research activities quickly intensified resulting with the fact that almost 20% of all the papers published in ArXiv during 2017 mention deep learning.
While this trend seems impressive enough, AI positions usually require polivalent individuals that have a broader set of competences beyond the theoretical understanding of AI concepts, since pure theoretical knowledge is not fully applicable without having strong programming skills, experience with data science practices and tools, understanding of statistics, and a dose of intuition. Besides in the tech industry, similar traits are also desirable in the financial, automotive, and pharmaceutical industries, to name just a few. The talent pool is shallow, so tech giants lure top experts with pay packages that other companies can rarely afford.
However, a multiperspective view on the question of AI talent shortage could offer some ideas for hiring in small companies and startups. The first perspective is related to varieties of AI jobs that are usually treated as only a few different positions with very broad roles, responsibilities, and expected competences. Building an AI-based product requires establishing the data infrastructure, selecting appropriate machine learning algorithms, preparing and understanding data, training and validating machine learning models, testing their performance, and developing the whole product around the algorithms. Those are fundamentally different activities that call for different skills, interests, and experiences.
Appropriately defined positions may bring a lot of benefits such as facilitated hiring process, improved expectation management, and more focused career development. Multiple job positions do not necessarily imply extreme specialization which could be a severe risk in quickly evolving environments. The scopes of different roles can overlap and constantly evolve to follow up with all the changes in the company.
An example of identifying and operalizing these differences is going beyond the usual division of Data Analyst, Data Scientist, and Data Engineering roles by separating research and operational or junior and senior positions. The purpose of this approach is to provide a framework that can help managers explain the expectations and hire the right people for the positions that are really needed. As an alternative to searching for rare extremely polivalent talents, well defined roles can guide the hiring process towards the optimal fit for the organization.
However, the granularity of AI roles should match the company needs, size, maturity, people, and technologies at the given moment. The challenging task for managers is finding the sweet spot between roles that are undefined, blurry, and confusing to people and those that are over-specialized, rigid, and too detailed, as the latter can negatively reflect on team’s abilities to innovate and diminish opportunities for individual growth. As always, a reasonable balance based on a multiperspective understanding should be the right way forward.
Building the knowledge within the company is another essential practice not just for alleviating the problem of talent shortage, but also for developing the excellence and ability to quickly react to changes in the market and technology. Many fast-paced startups use the syntagm “war time” as an excuse for constant hacking, and focusing on immediate or short-term results. However, a systematic and transparently supported competence buildup is a crucial approach to empower the company, especially in quickly developing fields such as AI.
By dedicating the time and budget for conferences, summer schools, online courses, books, and other types of educations, and by encouraging employees to join meetups, hackathons, and open-source projects, companies can play an important role in creating excellence and establishing a competitive advantage in the context of a talent shortage. Companies than can do this well are directly turning a big impediment into an opportunity which can eventually became a sustainable business model itself. The company where I work (Visage Technologies) employs a whole division to work as consultancy teams for an influential client on exciting AI products. This would not be possible if the company did not invest in education and (in my opinion very successfully) strive to be an exceptionally good place to work.
The final and perhaps the most far-reaching thought on talents important for AI startups is not about data scientists, machine learning experts, software engineers, or any other tech experts. It is about people whose contributions to building valuable products and utilizing technology to improve quality of life is equally important as technical contributions. They are UX researchers who provide valuable insights about target users, their habits, attitudes, behavior, and desires relying on psychological research methods; they are designers who decide how products should blend in existing habits and solve real problems, additionally enriching them with aesthetic values; they are marketing specialists who tell stories about products and companies.
While many jobs will be automated in the near future, defining the value and meaning is the fundamental anthropological characteristic that cannot be transferred to algorithms or machines, so the importance of people who systematically think about these topics can only increase over time. In Accenture’s research on re-configuring roles by AI, they identified leadership & management and empathy & support as two role clusters that will require a major increase in workers over the next decade. While Accenture’s research considers global trends across all industries, the conclusions evidently do not exclude tech companies.
All the discussed perspectives — appropriate AI job positions, systematic education of employees, and importance of non-engineering talents — are just a few of many topics related to better positioning of AI startups in the labor market. The reasons for focusing specifically on those topics are their relevance in the context of AI and the possible marginalization by startups in the “war time” that are fixated on short-term growth. As always, balance and reasonable prioritization of different practices including the usual hiring tricks (like building a strong employer brand and establishing relationships with universities to attract young talents) is a way to find an appropriate and authentic answer to the shortage of AI talents.
Access to Data
Another topic considered as a major impediment for AI startups is access to sufficient amounts of cleaned, structure, and labeled data. The very definition of machine learning emphasizes that progressive improvement to the algorithm’s performance on a specific task is achieved by learning from data in contrast to being explicitly programmed.
Relevant, sufficient in amount, and realistically distributed data are necessary for machine learning algorithms to capture important phenomena without overfitting to noise or outliers. It has been demonstrated a number of times how access to data propellered the pace of change in a specific field, even more vigorously than development of algorithms did. One such situation was the project ImageNet started in 2007 by Fei-Fei Li that played an important role in radical advancements of image recognition during the last decade.
More data do not only positively influence the development of AI systems, but also facilitate their testing and optimization. With over 130 million streaming subscribers worldwide, Netflix can take advantage of the large traffic volumes and run statistically meaningful A/B tests in order to compare different variations of recommendation algorithms or to understand which artworks attract more user attention. Comprehensive and extensive data sets for testing are even more important in safety-critical applications such as autonomous vehicles and robotics.
The means of data collection, labeling, and cleansing are specific to a particular context in terms of user data accessibility and size, data regulations, technologies for data collection, possibilities of generating artificial data, and other factors. Some companies like Netflix and Facebook rely on large amounts of data produced by their users, while other companies depend on their own expensive and quite demanding processes of data collection and labeling. Regardless of the selected approach, data collection is more accessible to larger companies with the established market position, capital, resources, and active users, because the processes of manual collection and annotation are characterized with high operating costs that are at best linearly dependent to data amounts, whilst user data harvesting requires a strong infrastructure and, more importantly, regularly engaged users.
However, instead of fatalistically struggling to implement a data collection process as quickly as possible to kick-off their business, AI startups should consider their data strategies in the longer term, taking into account some recent, more accessible approaches. An excellent example of how ambitious companies resonate about this topic is from the field of autonomous vehicles. Tesla and Waymo recognized that data collection strategies would make an important difference in the future success and they made strong moves, but took different approaches. With more than 300.000 cars sold worldwide, the main Tesla’s data sources seem to be customer-owned cars. Constrained by the 500 times smaller fleet, Waymo heavily relies on simulated data, but with a strong trend in gathering more and more miles on the public roads. It is inevitable that these two strategies will blend, as both of the companies will find their optimal combinations of simulated and real data.
The use of synthetic data in machine learning is an established practice that can facilitate development and testing in lab conditions. Some applications such as robotics and autonomous driving require extensive and quite sophisticated simulators for early-stage machine learning as well as for SiL (Software-in-the-Loop) and HiL (Hardware-in-the-Loop) testing. Several companies have readily tackled this problem, including NVIDIA with their well known DRIVE Constellation Simulation System, Siemens whose solution is a part of its Simcenter portfolio, and Microsoft working on projects such as Road Runner and the open source system for training drones, AirSim.
Since synthetic data may not be appropriate for all types of tasks and they cannot fully replace real data when it comes to production-ready AI solutions, startups still need some shortcuts to acquiring real data labeled for the purposes of supervised learning and performance evaluation. In many cases, manual labeling is cumbersome, as it requires proper tools, a lot of workforce, and coordination. For that reason, many companies recognized this emerging need as a business opportunity and started offering data annotation services and tools. Among the companies that hurried to win the leading positions in the forming value network, there are some big names like Amazon with thier Amazon Mechanical Turk (MTurk), but also a lot of startups like Scale API, Playment, and Gengo AI.
Labeling tools became semi-automated to disburden manual work and increase the quality of the labeled data. For example, labeling objects in visual sequences can be facilitated by mechanisms of visual tracking that automatically propagate annotations from a labeled frame to all following frames in which the same object appears. While AI startups can choose among a variety of options to kick-off their data labeling process, they also have an opportunity to join this race while the supply chain in the AI ecosystem is still in its early and fragile stage. Winning opportunistic positions in any part of the value network will be an important strategic move for tackling future challenges and achieving growth ambitions.
In order to improve the performance of machine learning algorithms in the context of hardly accessible or expensive labeled data, more and more practitioners find inspiration in approaches that emerged from academic research. Techniques like transfer learning and semi-supervised learning take advantage of data that otherwise do not seem useful for the specific task, in particular — labeled data developed for a different, but related task and large amounts of unlabelled data that complement smaller labeled datasets.
The technique of reusing a model developed for one task as the starting point of developing a model for another task is called transfer learning. It became a popular approach, especially in the context of deep learning, as it allows rapid progress in the learning process or improved performance of algorithm. An example of applying transfer learning is reusing parts of the model trained to detect general objects in images for the purpose of detecting diabetic retinopathy on retina images. Due to the existence of large high-quality datasets and pre-trained models, transfer learning is commonly used with image and language data. When a lot of unlabeled data is accessible along a smaller labeled dataset, semi-supervised learning techniques may be considered as a mechanism for improving performance. They are a class of supervised learning algorithms that make use of unlabelled data along with labeled data for training.
Although they require substantial efforts of AI experts in terms of carefully selected and designed features, models, kernels, and similarity functions, the aforementioned techniques may play an important role in achieving better accuracy with less additional investments in data collection and labeling. Synthetic data, simulators, semi-automated and outsourced data annotation, transfer learning, and semi-supervised learning are just some of the possible approaches to mitigate the lack of appropriate data. Besides the discussed topics, there are many other widely accepted approaches including harvesting free data sources and pre-labeled public datasets, augmenting labeled data sets, and relying on accessible pre-trained models.
While the problem of sufficient and quality data will stay an important question for AI companies, its formulation is changing over time, as it is tackled by more specialized companies and more advanced approaches. Those changes open new opportunities in the AI value network, and support startups in breaking the vicious circle more easily. Establishing the right data strategies from the early stages of the company’s life cycle may turn out to be a strong enabling force for efficient operations and competitive advantage as the company grows and the industry matures.
Value proposition as a success factor
While it may serve as an inspirational combination of insights that can help mitigating particular impediments, the discussion about talents and data just touched upon the manifestational aspects of the reasons why AI startups might have the lower chances of accessing disruption nowadays. The roots may be less specific to AI and more related to the contrast of sustaining and disruptive changes formulated by Clayton M. Christensen in his book The Innovator’s Dilemma that became one of the most influential business bestsellers. The author described sustaining technologies as those that “improve the performance of established products, along the dimensions […] that mainstream customers in the major markets have historically valued” no matter whether changes are radical or just incremental.
On the other hand, disruptive technologies bring a different value proposition and generally result in worse product performance in mainstream markets, at least in short terms. As shown by Christensen’s research and multiple case studies, disruptive technologies are those that create chances for entrants to overtake leading companies. A changed value proposition typically entails simpler and cheaper products that are first commercialized in emerging or marginalized markets and embraced by at least profitable customers. However, in the course of time, such products enhance their performance following the trajectories of sustainable technological improvements and arrive to the position to attack the mainstream market.
The radicality of AI as a technology can be easily misinterpreted as the disruptive potential of AI products. An excellent example is Smart Compose feature in Gmail presented at Google I/O in May 2018 that suggests contextually relevant completion of sentences while typing an email. As a natural extension of Smart Reply feature, it drastically improves the experience of writing emails by saving time and reducing the chance of grammatical and spelling errors. Such an advanced feature based on complex technology inevitably steeply raises Gmail along the curve of technological improvement.
However, it is not a disruptive change, as it improves the established product within the historically valued context, does not target a new market, or changes a value proposal of the product. If a startup developed this feature within their emerging e-mail client, it would not represent a threat for Google, since the tech giant would easily recognize that the improvement fits the needs of their customers and simply adopt the feature or buy the startup. Leading companies are not threatened by sustainable improvements because they are already good at what they are doing and can easily extend their products in the direction of established values, due to the deep understanding of their users and strong market position.
While sustainable improvements do not open much space for entrants, they are correlated with the raising performance demand of the market. Users of LinkedIn messaging could access the Smart Reply feature just several months after it appeared in Gmail. Although Smart Reply and Smart Compose are useful, radical, and amazing improvements that can enhance user engagement and even contribute in attracting new users, they do not alter the value proposition. Therefore, such features will not lead entrants towards disrupting the well-established mainstream markets.
Recognizing whether a certain technological improvement is sustainable or disruptive is not always that straightforward. Less than a couple of years ago, the emerging chatbot revolution ushered in great expectations and disruption became a buzzword that was almost inseparable from chatbots. However, in contrast to all overly optimistic predictions, the disruption still did not happen. One component of assessing the disruptive potential is to observe trajectories of the performance improvement demanded by the market versus trajectories of the performance improvement that chatbots may provide. If chatbots improve faster than demanded in the market, they will have a chance for disruption.
This analysis cannot be conducted superficially, as identifying the relevant axes of improvement trajectories is difficult. Currently, many chatbot critics argue that advances in AI constraint the improvement trajectory for chatbots. The technology is not there yet, and chatbots cannot provide acceptable user experience without excessive human help. Whether this is true or not, the state of AI-powered conversational technology is not the only dimension to observe. The notion of chatbots as a disruptive force is highly multidimensional, so all the elements of its value proposition should be considered. Even with the technology perfected, it remains unknown whether users will embrace the conversational interfaces as the means of interaction with AI systems, what markets are more prone to the disruption, and how scalable technology may become. The difficulty of these questions entails the difficulty of predicting the timing and exact markets where the disruption may occur.
The value proposition is and has always been the single most important success factor for startups. Applying AI in the direction of existing advancements in industries that have undergone a lucrative digital transformation during the last two decades may work for the leading companies, but it is surely not a formula for disruption. Instead of repeating the business models from technologies that passed their disruptive phases, AI startups should aim at formulating their authentic value proposals, primarily inspired by the genuine desire to improve the quality of life for individuals and communities — even for those that do not seem to fit the most profitable market segment. The multiperspective view composed of particular observations about industry trends, the scarcity of talents, access to data, and the question of value proposal should serve as a constructive and encouraging stimulus for building a unique path towards valuable products.