Medicine Under the Magnifying Glass
We’ve moved from a beloved old adage to formal characterizations of systemic problems; from models that describe the unreliability of research environments and the impermanence of facts, to direct measures of medical reversals and under-evidenced interventions. We’ve reviewed the aggregate impact of medicine to consider the implication that, if a significant portion of treatments are ineffective, then we would see only a modest net benefit from medicine. And this is indeed what researchers find.
To restate, “How many established standards of medical care are wrong? It is not known.” But disturbingly, the estimate of half wrong cannot be dismissed as mere hyperbole.
If science has taken a turn towards darkness, efforts in meta research should be celebrated as beacons of light. But it’s not enough.
Hanson calls for action. “We already knew some medicine is more helpful and some more harmful than average. It doesn’t really help to know which part is which unless we are willing to somehow act on that information, and treat better parts differently from worse parts.” Ioannidis is similarly dissatisfied with problems. “My wish is not to expose problems. Obviously, there are lots of problems, so it is not a big deal to point out another one, but there is no end to them. My wish is to try to fix problems.”
In this spirit we turn to artificial intelligence. Is AI submerged in the problem, amplifying both good and bad medicine? Or might the AI community join the contrarians, disrupting medicine from the outside?
Part 2: Meta research and artificial intelligence
“It’s hard to be a disruptor in this area” David Moher
As Ioannidis highlighted in a recent interview with Eric Topol, the potential for meta research is astounding. “One-percent improvement because of adopting a better scientific process across science is tremendous progress. It could translate to tens of millions of lives saved.” Given the trillion dollar scale of healthcare and the proportion of questionable medical practices, it could also save billions.

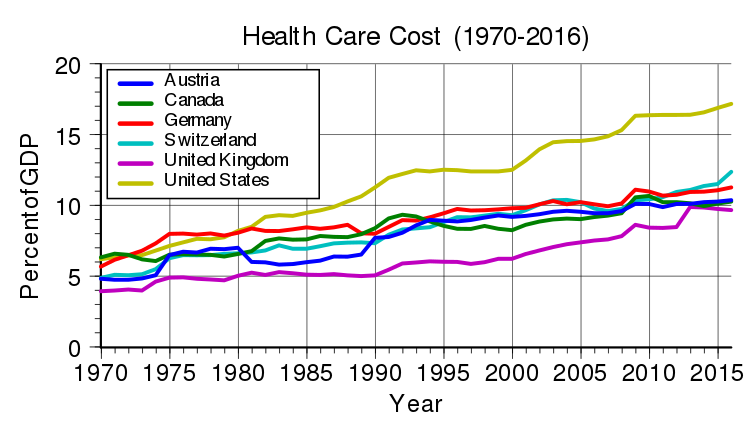
Do these lives and riches translate to substantial investment in meta research? Unfortunately, not yet.
Meta research as a field
As reported by Jennifer Couzin-Frankel in Science, funding for people working within meta research is scarce. “If you approach government funders, foundations, often they’re asking what diseases you’re curing,” says An-Wen Chan, a skin cancer surgeon and scientist at Women’s College Research Institute in Toronto. Funding is typically organized along topical lines, not cross-disciplinary methods. “Research in this area is not fast-moving,” says Sara Schroter, a senior researcher at The BMJ. “It’s hard to be a disruptor in this area.” according to David Moher, a clinical epidemiologist at The Ottawa Hospital Research Institute.
In one sense, efforts in meta research are ubiquitous. Scientists from all fields are continually honing their methods and processes. But these efforts are also highly fragmented. Coordination and knowledge-sharing is limited.
In their review of the meta-research field, Ioannidis and his colleagues called for a more unified effort. “A research effort is needed that cuts across all disciplines, drawing from a wide range of methodologies and theoretical frameworks, and yet shares a common objective; that of helping science progress faster by conducting scientific research on research itself.”


Dominant categories of AI investment
Meta researchers are increasingly using AI and machine learning techniques to increase their productivity. A notable example is the recent work of Thomas Stoeger and his colleagues at Northwestern University. They investigated why biomedical research focuses on a minority of all known genes. “Our work demonstrates that even highly promising genes that could already be studied by current technologies remain ignored.”
Their research drew international headlines, highlighting a problem that would otherwise fester in darkness. Such is the promise of AI as a searchlight for meta research.
But meta research has not yet been widely embraced by the AI community at large. The dominant categories are focused on applications within the existing medical and healthcare complex.
According to principal analyst Keith Kirkpatrick of the market intelligence firm Tractica, “Cost reduction is a major driver of many healthcare initiatives and incorporating AI technology is no exception. AI applications are designed to address specific, real-world use cases that make the diagnosis, monitoring, and treatment of patients more efficient, accurate, and available to populations around the world.”
While ROI and cost reduction drive investment, it’s hard to connect the dots in meta research, from abstract processes and methodologies to the massive costs of over-diagnosis and over-treatment.
Ironically, most of the AI community is focusing its productivity punch in areas that are already being criticized for moving too fast. While medicine is accelerating, so is the prevalence of under-evidenced treatments. This is hardly surprising, given that only a small fraction of funding is directed to treatment evaluation (less than 10%, based on a 2006 review of UK health research funding).
AI is mirroring existing investment patterns, incentivized to produce more medicine at lower costs. If data is the new oil, too much medicine is runaway climate change.
And the window of opportunity may be closing. The applications for AI in healthcare have been “proven useful” and are thus crystallizing. Startups and investors are defining the product-market boundaries for AI and healthcare, as a necessary precursor to commercialization. While their optimism and commitment are cause for celebration, it’s also important to note that these categories establish not only what will be pursued, but also the areas at risk of neglect.
Is AI is necessarily good for medicine?
Some might argue that all efforts in AI tackle the problem of bad medicine. There’s a widespread belief that knowledge can be derived from data, and that machines can overcome the inherent biases and failings of human decision-makers. If true, bad medicine isn’t an impediment to AI, it’s what makes AI necessary.
It’s a big question, so I’ll return to it in a future post. For now, suffice it to say skepticism is warranted.
Garbage in, garbage out is a principle of computing as old as Charles Babbage. As discussed in a previous post, the main challenge with today’s AI concerns this very problem of how to manage the problem of induction to create good knowledge. Inductive methods require sound explanations and background knowledge to work effectively. There is no universal learner that works well in all environments, so existing domain knowledge is an essential factor.
And there’s no shortage of contemporary examples of algorithms amplifying bad choices, from racist chatbots, to discriminating recruiting systems, to unethical assessments in legal proceedings.
AI offers no all-purpose cure. A fundamental problem like bad medicine needs targeted solutions.
A new calling for AI
In his 2016 confession to David Sackett, Ioannidis offered a hand-wringing testimony of his experiences in clinical research. “David, I am a failure.” He described a deteriorating problem. “The GDP devoted to health care is increasing, spurious trials and even more spurious meta-analyses are published at a geometrically increasing pace, conflicted guidelines are more influential than ever, spurious risk factors are alive and well, quacks have become even more obnoxious, and approximately 85% of biomedical research is wasted.”
“David, I am a failure” John Ioannidis
It’s painful to hear champions like Ioannidis describing their efforts as failures. It takes courage to tackle this problem from within. But the existing incentive structures in healthcare make meta research an uphill battle for incumbents.
This is why meta research needs more attention from startups, technologists, and investors.
Healthcare is an inhospitable environment for innovation under the best of circumstances. Those that choose to innovate in this area are trying to make a difference, to save lives and improve human well-being. The data scientist, Benjamin Rogojan, captures the spirit of this higher purpose well. “As healthcare professionals and data specialists, we have an obligation not just to help our company, but to consider the patient. We need to not just be data driven but be human-driven.”
Meta research offers the AI community a powerful opportunity to be human-driven. It embraces perhaps the biggest problem in healthcare. “Move fast and break things” doesn’t play well in medicine, but this is the meta realm. Meta research needs your speed. It needs your urgency. It not only welcomes disruption, it can’t succeed without it.