AI Ethics and the Race to Bring Pen & Paper Industries Online
AI Ethics and the Race to Bring Pen & Paper Industries Online
A Conversation with Leo Polovets of Susa Ventures

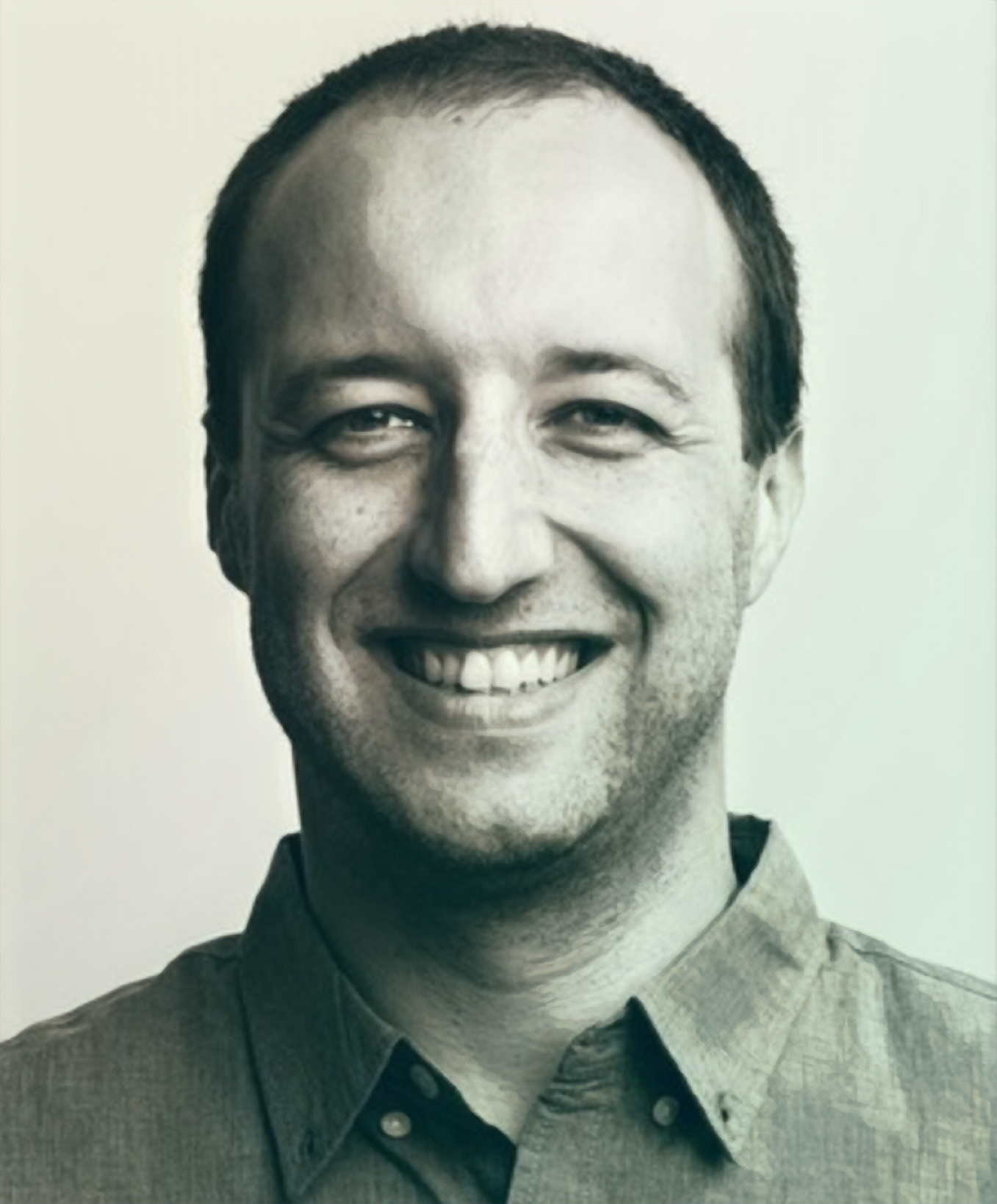
Leo Polovets of Susa Ventures recently sat down with Machine Learnings for a chat about machine learning, data moats, and artificial intelligence ethics.
Sam: Leo! It’s great to meet you.
Can you introduce yourself and context for the work you’ve done that our audience may be unaware of?
Leo: It’s nice to meet you too, Sam. Sure.
I’m 1 of 3 partners at a $50 million seed fund called Susa Ventures. I’ve invested in companies like Flexport, Robinhood, and LendUp to name a few. Before the seed fund, I’d been a software engineer for a decade — including at LinkedIn back when they were 13 employees.
After that I went to Google where I got my first exposure to Big Data and machine learning around fraud detection.
My team was responsible for processing incoming checkout transactions and predicting fraud in real time for a product called Google Checkout. It shut down a few years ago, and was rolled into Google Wallet.
After that I found myself missing startup life, so I went to a location data startup called Factual where I dabbled mostly in data processing, cleaning, and de-duping.
I haven’t done machine learning coding hands-on, but I’ve been involved in a number of projects where it was leveraged.
Sam: Awesome. You’ve written about software commoditization and the importance of building large, unique datasets. One of the topics we enjoy covering most in Machine Learnings is the ways in which startups are building data moats.
In your opinion, what are the most interesting data moats being built today?
Leo: The most interesting moats are being built by vertical SaaS products in industries that’ve only been lightly touched by Tech — tools for lawyers, logistics, shipping, and things like that.
The products are so interesting because software hasn’t fully permeated these industries. The startups disrupting these industries will typically start with a minimum viable product that doesn’t require machine learning at all.
The product will be more of a workflow tool that helps the end user do something with an app or tablet that they’ve historically done with pen and paper. People will use the new product really just for the added speed and convenience. In the background, the company is building a dataset that no one has ever had in digital form, and can start layering machine learning on top of that dataset over time.
An example of this is a company called SimpleLegal. They make a system of record for corporate legal departments, which work heavily with external lawyers.
Part of the job of a legal department is billing management and approval, as well as budgeting — basically figuring out where the money is going. Up to now, the job entailed lawyers sending 120-page physical documents back and forth, and it’s utter hell.
SimpleLegal brings that process online, and accepts documents in a more structured way. For the legal departments, this structure translates to less headaches and money spent.
For SimpleLegal, the structured data helps them build an understanding of how much tasks costs across companies, how different firms bill, and to automate auditing for their customers.
They can identify if a particular law firm keeps billing for things they shouldn’t, which firms have the best results, and ultimately the data can be used to helps companies make better legal decisions. With bill rates for partners regularly exceeding $1000/hr, billing problems can really add up. This is where applying machine learning becomes a very interesting — and valuable — proposition.
Sam: Fascinating. You mentioned earlier that the shipping industry is another Pen and Paper industry that’s ripe for disruption?
Leo: Absolutely. I invested in the logistics space through Flexport, which modernizes freight forwarding.
They help companies move goods internationally. Shipping a container of goods involves a lot of steps, including a truck on both sides of the ocean, a ship in the middle, customs forms, insurance, and so on. All of the moving pieces involved in the process make it incredibly painful to figure out the associated costs and to have predictable shipping schedules.
Freight forwarders help coordinate all of these steps, but traditionally work using a combination of voicemail, email, and fax. Flexport brings this process online, and they’re accumulating enough data to give real-time price quotes, help optimize shipping providers, and deliver a much better customer experience, locking out competitors from the space.
Sam: Okay let’s switch gears. There are obvious benefits to bringing these processes and datasets online — but I doubt this will always be for the good.
For example, earlier this year the Presidential Advisory Commission on Election integrity sent out a request for voter roll data (name, address, dob, political party, voter history, SSN) from states to “fully analyze vulnerabilities and issues related to voter registration and voting.” One doesn’t have to think too hard to imagine how this data could be abused to suppress voting from specific populations.
Now that machine learning allows us to process and draw connections between ever larger data sets, how should developers decide which ones are ethical to work on?
Leo: To be honest I don’t have a good answer for this.
Everyone has their own code of ethics, and individuals will have to make the personal call as to whether or not they’re okay with the potential impact of the algorithms they build.
Technology is like science in many ways. It has the potential for good. It has the potential for evil.
You can use Google to diagnose if you’re having a heart attack, or to find instructions on bomb making.
It’s up to people building products to think critically about the societal and ethical implications of their work. It’s the unfortunate truth that many things built with the best intentions can be abused for evil. It’s a tough question.
Sam: Leo, thanks so much.
Leo: It was fun to chat, Sam.