Machine Learning Moves Into Fab And Mask Shop
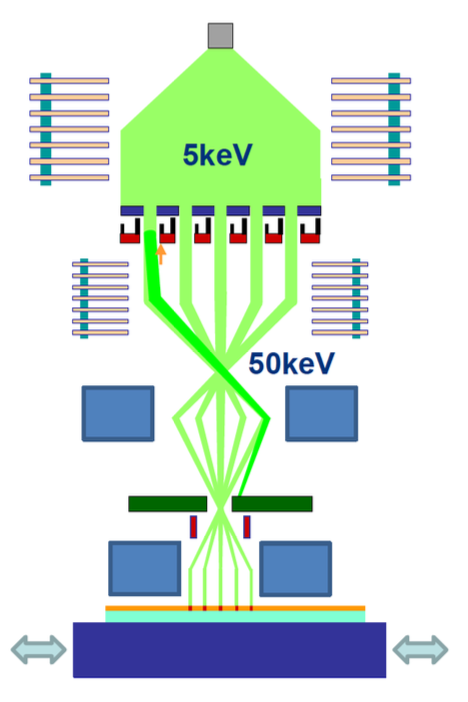
Semiconductor Engineering sat down to discuss artificial intelligence (AI), machine learning, and chip and photomask manufacturing technologies with Aki Fujimura, chief executive of D2S; Jerry Chen, business and ecosystem development manager at Nvidia; Noriaki Nakayamada, senior technologist at NuFlare; and Mikael Wahlsten, director and product area manager at Mycronic. What follows are excerpts of that conversation.
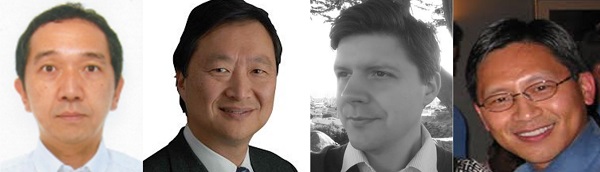
SE: Artificial neural networks, the precursor of machine learning, was a hot topic in the 1980s. In neural networks, a system crunches data and identifies patterns. It matches certain patterns and learns which of those attributes are important. Why didn’t this technology take off the first time around?
Chen: There are three reasons. One is that these models are so powerful. They need to consume a lot of data. It is pulling out latent information in a lot of data, but it needs the fuel to enable deep learning. The data is like the fuel. You need enough data to feed this powerful model. Otherwise, the model absorbs too much information and it becomes overtrained. We haven’t really had access to data until more recently.
The second thing is that there has been some evolution in the mathematical techniques and the tools. I’ll call that algorithms and software, and there is a big community now that is building things like TensorFlow and such. Even Nvidia is contributing a lot of the lower-level software, as well as some higher-level software that makes it easier and more accessible for the world.
The last piece of that, of course, is very dense and inexpensive computing. All of that data needs to be digested by all these fancy algorithms. But you need to do it in a practical lifetime, so you need this dense computing in order to process all of that data and come up with a solution or some kind of representation on what the world looks like. Then, you combine that, of course, with these physics models, which themselves are also very compute-intensive. This is why a lot of these national supercomputer facilities like the Summit machine at Oak Ridge National Laboratory are built with tons of GPUs, almost 30,000 GPUs. That’s the driving force. They recognize that these two sides of the coin, physics-based computing and data-driven computing, especially deep learning, are necessary.
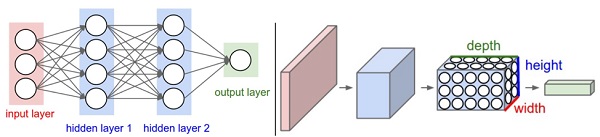
SE: Google, Facebook and others make use of machine learning. Who else is deploying this technology?
Chen: It’s pretty much every vertical industry. It’s every company that we would consider as a cloud service provider. So they are either providing cloud infrastructure or they are providing services available from the cloud like a Siri or Google Voice. It’s no secret that they are doing a lot of this stuff. More recently, we’ve seen that technology migrate out into specific vertical applications. Obviously, there has been a lot of activity in a variety of healthcare spaces. These spaces have traditionally used lots of GPUs for visualization and graphics. Now, they are using AI to interpret more of the data they obtain, especially for radiological images in 3D volumes. We see it happening in finance, retail and telecommunications. We see lots of applications for these industrial, capital-intensive types of businesses. The semiconductor industry is the one that is the most obvious and active. The timing is perfect, and there is a lot of progress that we can make here.
Fujimura: Medical is an example. In medical imaging, you are really honing in on exactly which cells are cancerous. Using a deep learning engine, they can actually narrow it down to exactly which cells are bad. That’s a medical example. But you can imagine the same benefits that can be derived in semiconductor production.
SE: It appears that machine learning is moving into the photomask shop and fab. We’ve seen companies begin to use the technology for circuit simulation, inspection and design-for-manufacturing. I’ve heard machine learning is being used to make predictions on problematic areas or hotspots. What is the purpose here or what does this accomplish?
Fujimura: A traditional hotspot detector requires a whole series of filters. A human can’t possibly go through or even 100 humans can’t go through an entire mask or whatever and find these places. It can focus on suspect areas only. Once a machine learns to do it through deep learning, it will just diligently go through everything. So it’s going to be more accurate.
Chen: On many of the industrial applications, sometimes in the end the accuracy may be as comparable for a human. But there are two sides to accuracy. There is the detection part of it, but there is also the false positive side of it. This is maybe more prevalent in other businesses than semiconductors, although it’s also prevalent in semiconductors. The cost of dialing up your sensitivity to make sure you detect everything might be that you generate a huge pile of false positives. That can be very costly. But there is also a lot of evidence and research that show that deep learning solutions are able to, in many cases, achieve comparable or better sensitivity, while not causing the burden of a lot of false positives.