Boosting Sales With Machine Learning


Boosting Sales With Machine Learning
How we use natural language processing to qualify leads
In this blog post I’ll explain how we’re making our sales process at Xeneta more effective by training a machine learning algorithm to predict the quality of our leads based upon their company descriptions.
Head over to
The problem
It started with a request from business development representative Edvard, who was tired of performing the tedious task of going through big excel sheets filled with company names, trying to identify which ones we ought to contact.

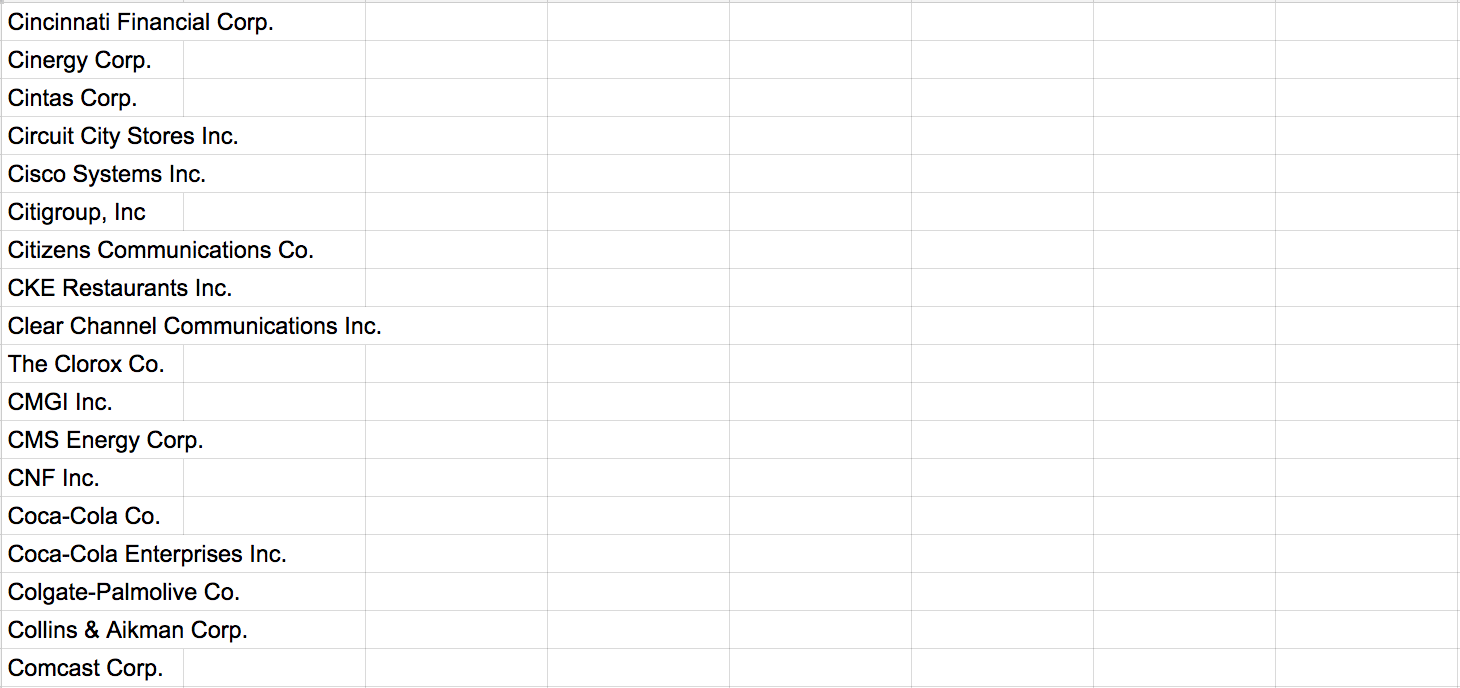
This kind ofpre-qualification of sales leadscan take hours, as it forces the sales representative to figure out what every single company does (e.g. through read about them on LinkedIn) so that he/she can do a qualified guess at whether or not the company is a good fit for our SaaS app.
And how do you make a qualified guess? To understand that, you’ll first need to know what we do:
In essence, Xeneta help companies that ship containers discover saving potential by providing sea freight market intelligence.

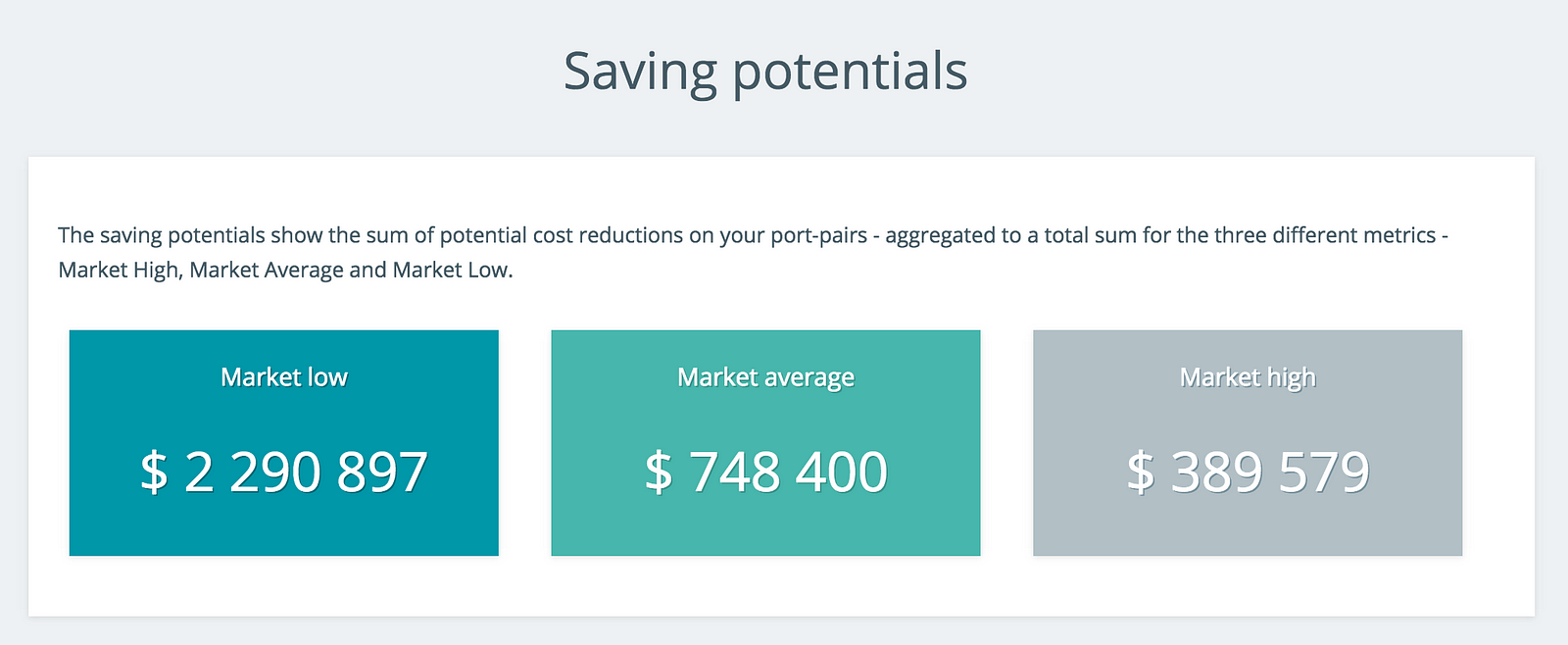
More specifically, if your company ships above 500 containers per year, you’re likely to discover significant saving potential by using Xeneta, as we’re able to tell you exactly where you’re paying above the market average price.

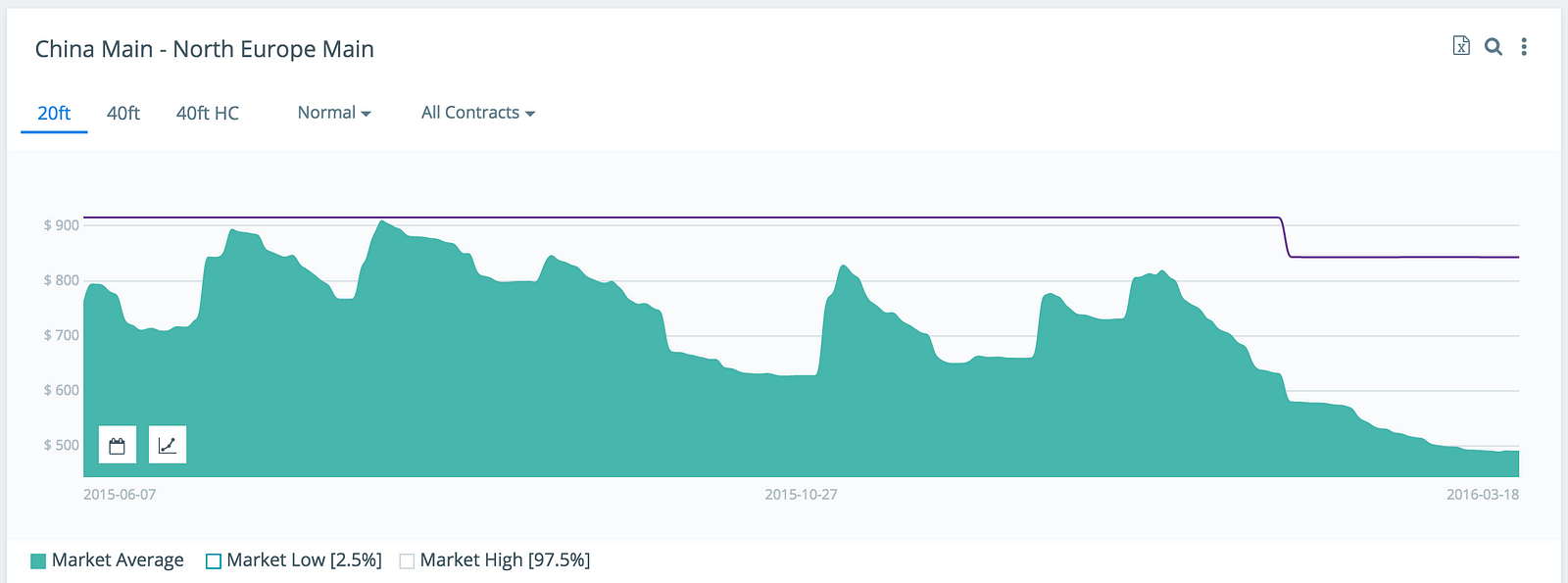
This means that our target customers are vastly different from each other, as their only common denominator is that they’re somewhat involved in sea freight. Here are some examples of company categories we target:
- Automotive
- Freight forwarding
- Chemicals
- Consumer & Retail
- Low paying commodities
The hypothesis
Though the broad range of customers represents a challenge when finding leads, we’re normally able to tell if a company is of interest for Xeneta by reading their company description, as it often contains hints of whether or not they’re involved in sending stuff around the world.
This made us think:
Given a company description, can we train an algorithm to predict whether or not it’s a potential Xeneta customer?
If so, this algorithm could prove as a huge time saver for the sales team, as it could roughly sort the excel sheets before they start qualifying the leads manually.
The development
As I started working on this, I quickly realised that the machine learning part wasn’t be the only problem. We also needed a way to get hold of the company descriptions.
We considered crawling the companies’ websites and fetch the About us section. But this smelled like a messy, unpredictable and time consuming activity, so we started looking for API’s to use instead. After some searching we discovered FullContact, which have a Company API that provides you with descriptions of millions of companies.


However, their API only accept company URL’s as inputs, which rarely are present in our excel sheets.
So we had to find a way to obtain the URL’s as well, which made us land on the following workflow:
- Using the Google API to google the company name (hacky, I know…)
- Loop through the search result and find the most likely correct URL
- Use this URL to query the FullContact API
There’s of course a loss at each step here, so we’re going to find a better way of doing this. However, this worked well enough to test the idea out.
The dataset
Having these scripts in place, the next step was to create our training dataset. It needed to contain at least 1000 qualified companies and 1000 disqualified companies.
The first category was easy, as we could simply export a list of 1000 Xeneta users from SalesForce.
Finding 1000 disqualified was a bit tougher though, as we don’t keep track of the companies we’ve avoided contacting. So Edvard manually disqualified 1000 companies.
Cleaning the data
With that done, it was time to start writing the natural language processing script, with step one being to clean up the descriptions, as they are quite dirty and contain a lot of irrelevant information.
In the examples below, I’ll go though each of the cleaning techniques we’re currently applying, and show you how a raw description ends up as an array of numbers.

RegExp
The first thing we do is to use regular expressions to get rid non-alphabetical characters, as our model will only be able to learn words.
description = re.sub(“[^a-zA-Z]”, “ “, description)

Stemmer
We also stem the words. This means reducing multiple variations of the same word to its stem. So instead of accepting words like manufacturer, manufaction, manufactured & manufactoring, we rather simplify them to manufact.
from nltk.stem.snowball import SnowballStemmer
stemmer = SnowballStemmer(‘english’)
description = getDescription()
description = [stemmer.stem(word) for word in description]

Stop words
We then remove stop words, using Natural Language Toolkit. Stop words are words that have little relevance for the conceptual understanding the text, such as is, to, for, at, I, it etc.
from nltk.corpus import stopwords
stopWords = set(stopwords.words('english'))
description = getDescription()
description = [word for word in description if not word in stopWords]

Transforming the data
But cleaning and stemming the data won’t actually help us do any machine learning, as we also need to transform the descriptions into something the machine understands, which is numbers.
Bag of Words
For this, we’re using the Bag of Words (BoW) approach. If you’re not familiar with BoW, I’d recommend you to read this Kaggle tutorial.
BoW is a simple technique to turn text phrases into vectors, where each item in the vectors represents a specific word. Scikit learn’s CountVectorizer gives you super simple way to do this:
from sklearn.feature_extraction.text import CountVectorizer
vectorizer = CountVectorizer(analyzer = ‘word’, max_features=5000)
vectorizer.fit(training_data)
vectorized_training_data = vectorizer.transform(training_data)
The max_features parameter tells the vectorizer how many words you want to have in our vocabulary. In this example, the vectorizer will include the 5000 words that occur most frequently in our dataset and reject the rest of them.

Tf-idf Transformation
Finally, we also apply a tf-idf transformation, which is a short for term frequency inverse document frequency. It’s a technique that adjusts the importance of the different words in your documents.
More specifically, tf-idf will emphasise words that occur frequently in a description (term frequency), while de-emphasise words that occur frequently in the entire dataset (inverse document frequency).
from sklearn.feature_extraction.text import TfidfTransformer
tfidf = TfidfTransformer(norm=’l1')
tfidf.fit(vectorized_training_data)
tfidf_vectorized_data = tfidf.transform(vectorized_training_data)
Again, scikit learn saves the day by providing tf-idf out of the box. Simply fit the model to your vectorized training data, and then use the transform method to transform it.


The algorithm
After all the data has been cleaned, vectorised and transformed, we can finally start doing some machine learning, which is one of the simplest parts of this task.
I first sliced the data into 70% training data and 30% testing data, and then started off with two scikit learn algorithms: Random Forest (RF) and K Nearest Neighbors (KNN). It quickly became clear that RF outperformed KNN, as the former quickly reached more than 80% accuracy while the latter stayed at 60%.
Fitting a scikit learn model is super simple:
def runForest(X_train, X_test, Y_train, Y_test):
forest = RandomForestClassifier(n_estimators=100)
forest = forest.fit(X_train, Y_train)
score = forest.score(X_test, Y_test)
return score
forest_score = runForest(X_train, X_test, Y_train, Y_test)
So I continued with RF to see how much I could increase the accuracy by tuning the following parameters:
- Vocabulary: how many words the CountVectorizer includes in the vocabulary (currently 5K)
- Gram Range: size of phrases to include in Bag Of Words (currently 1–3, meaning up until ‘3 word’-phrases)
- Estimators: amount of estimators to include in Random Forest (currently 90)
With these parameters tuned, the algorithm reaches an accuracy of 86,4% on the testing dataset, and is actually starting to become useful for our sales team.
The road ahead
However, the script is by no means finished. There are tons of way to improve it. For example, the algorithm is likely to be biased towards the kind of descriptions we currently have in our training data. This might become a performance bottle neck when testing it on more real world data.
Here are a few activities we’re considering to do in the road ahead:
- Get more data (scraping, other API’s, improve data cleaning)
- Test other types of data transformation(e.g. word2vec)
- Test other ml algorithms (e.g. neural nets)
We’ll be pushing to GitHub regularly if you want to follow the progress. And feel free to leave a comment below if you have anything you’d like to add.
Cheers,
相關推薦
Boosting Sales With Machine Learning
Boosting Sales With Machine LearningHow we use natural language processing to qualify leadsIn this blog post I’ll explain how we’re making our sales proces
[Javascript] Classify text into categories with machine learning in Natural
bus easy ann etc hms scrip steps spam not In this lesson, we will learn how to train a Naive Bayes classifier or a Logistic Regression cl
[Javascript] Classify JSON text data with machine learning in Natural
comm about cnblogs ++ get ssi learn clas save In this lesson, we will learn how to train a Naive Bayes classifier and a Logistic Regressi
Classification and inference with machine learning
machine learning作業代寫、代做Markdown留學生作業、代寫python, C/C++程式語言作業Project 3Classification and inference with machine learningThis notebook is arranged in cells. Te
Minimizing Train Delays with Machine Learning
Machine learning can improve rail travel both in the long and the short-term by minimizing train delays and ensuring high service quality. Train delays can
Customer Segmentation with Machine Learning
on items that are frequently bought in bulk, such as pens and notepads for office supplies, is likely to make bulk buyers log in to the online store and
Convert unstructured data to structured data with machine learning
They stream movies and send texts and pictures to the other side of the world. Each second, a huge amount of data is created and collected. But, still, bus
Personalization at Scale With Machine Learning: The Xero Story
When Nigel Piper, Executive General Manager, first joined Xero, the company only had 100,000 subscribers. In over ten years that number has risen to over 1
step Time Series Forecasting with Machine Learning for Household Electricity Consumption
Given the rise of smart electricity meters and the wide adoption of electricity generation technology like solar panels, there is a wealth of electricity
CAD: Painting a Clearer Picture of the Heart with Machine Learning
Coronary Artery Disease (CAD) is a condition in which plaque forms on the walls of coronary arteries, causing them to narrow. Eventually, this could lead t
Quantum computers tackle big data with machine learning
WEST LAFAYETTE, Ind. -- Every two seconds, sensors measuring the United States' electrical grid collect 3 petabytes of data – the equivalent of 3 million g
Microsoft Releases ML.NET 0.6 with Machine Learning APIs
Earlier this year, Microsoft launched ML.NET, a cross-platform machine learning platform for .NET developers. This week, the company is rolling out ML.NET
Studying the stars with machine learning
Kevin Schawinski had a problem. In 2007 he was an astrophysicist at Oxford University and hard at work reviewing seven years' worth of photographs from the
Cool Factor: How to Steal Styles with Machine Learning, Turi Create, and ResNet
Turi Style TransferFirst of all, follow the Turi Create installation instructions on GitHub. It’s imperative to create a Python 2.7 environment with the sp
Getting Started With Machine Learning
Getting Started With Machine LearningWhat are the fundamentals of machine learning, and what are the necessary tools to evaluate risk and other concerns in
Educating the next generation of medical professionals with machine learning is essential
"The general public has become quite aware of AI and the impact it can have on health care outcomes such as providing clinicians with improved diagnostics
The Problem With Machine Learning In Healthcare
Recently an article by the Wall Street Journal has been floating around online that discussed how models will run the world. I believe there is a lot of tr
Android Developers Blog: Combating Potentially Harmful Applications with Machine Learning at Google: Datasets and Models
Posted by Mo Yu, Damien Octeau, and Chuangang Ren, Android Security & Privacy Team In a previous blog post, we talked about using machine learnin
Balancing CartPole with Machine Learning
Learn how to balance a CartPole using machine learning in this article by Sean Saito, the youngest ever Machine Learning Developer at SAP and the first
Start Here With Machine Learning
Need Help Getting Started with Applied Machine Learning? These are the Step-by-Step Guides that You’ve Been Looking For! What do you want help with?