GraphQL: A success story for PayPal Checkout
Our API journey
PayPal’s Checkout products spread across many web and mobile apps, supporting millions of users across ~200 countries and has hundreds of experiments running at any time. These apps leverage the same suite of REST APIs to fetch data needed for building UI.
REST
About 4 years ago, we went all in on
However, REST’s principles don’t consider the needs of Web and Mobile apps and their users. This is especially true in an optimized transaction like Checkout. Users want to complete their checkout as fast as possible. If your applications are consuming atomic REST APIs, you’re often making many round trips from the client to the server to fetch data. With Checkout, we’ve found that every round trip costs at least 700ms in network time (at the 99th percentile), not counting the time processing the request on the server. Every round trip results in slower rendering time, more user frustration and lower Checkout conversion. Needless to say, round trips are evil!
Sure, you can build an orchestration API to return all the data you need, but that comes with trade-offs. What do you name it? GET /landing-page? Now this seems like a JSON API, not a REST API. With orchestration APIs, your clients are coupled to your server. Any time you add a new feature or experiment, you add it to your API. Now you’re
When new requirements come along, developers face a choice: Should we create a new endpoint and have our clients make another request for that data? Or should we overload an existing endpoint with more data?
Developers often choose the 2nd option because it’s easier to add another field and prevent a client-to-server round trip. Over time, this causes your API to become heavy, kludgy, and serve more than a single responsibility.


We started with great intentions. Our API returned user information, a shipping address and funding options. Everything you need to build a Checkout app. Over time, use cases pile up. Every user pays the cost of these fields even if they don’t need them.


Orchestration APIs seem great at first, but we always regret them later.
Batch REST
REST wasn’t working for us, so we built a Batch REST API that solves some of these problems. It’s an on-the-fly orchestration API that allows clients to determine the size and shape of the response. Batch allowed us to combine atomic REST requests and reduce round trips.
Here’s an example of a Batch request and response. The request contains a map of REST operations where you specify verbs, endpoints, parameters and dependencies between them.

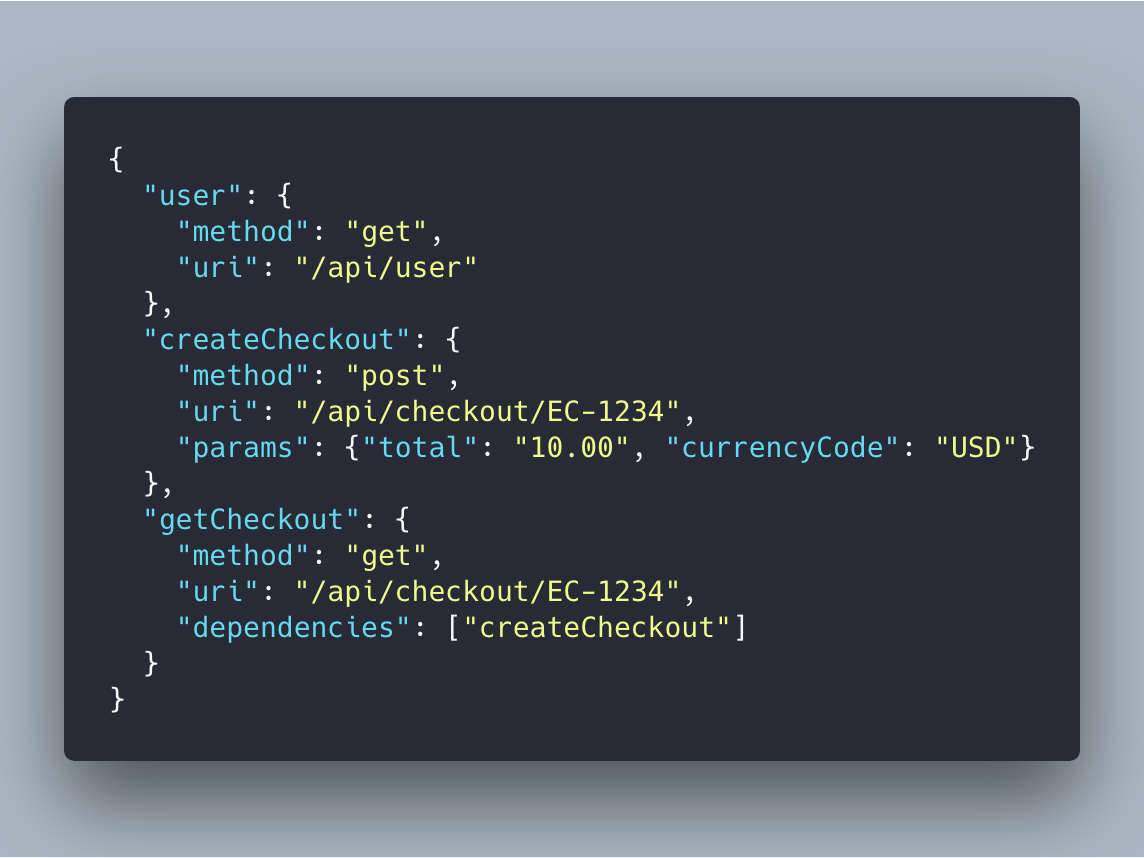

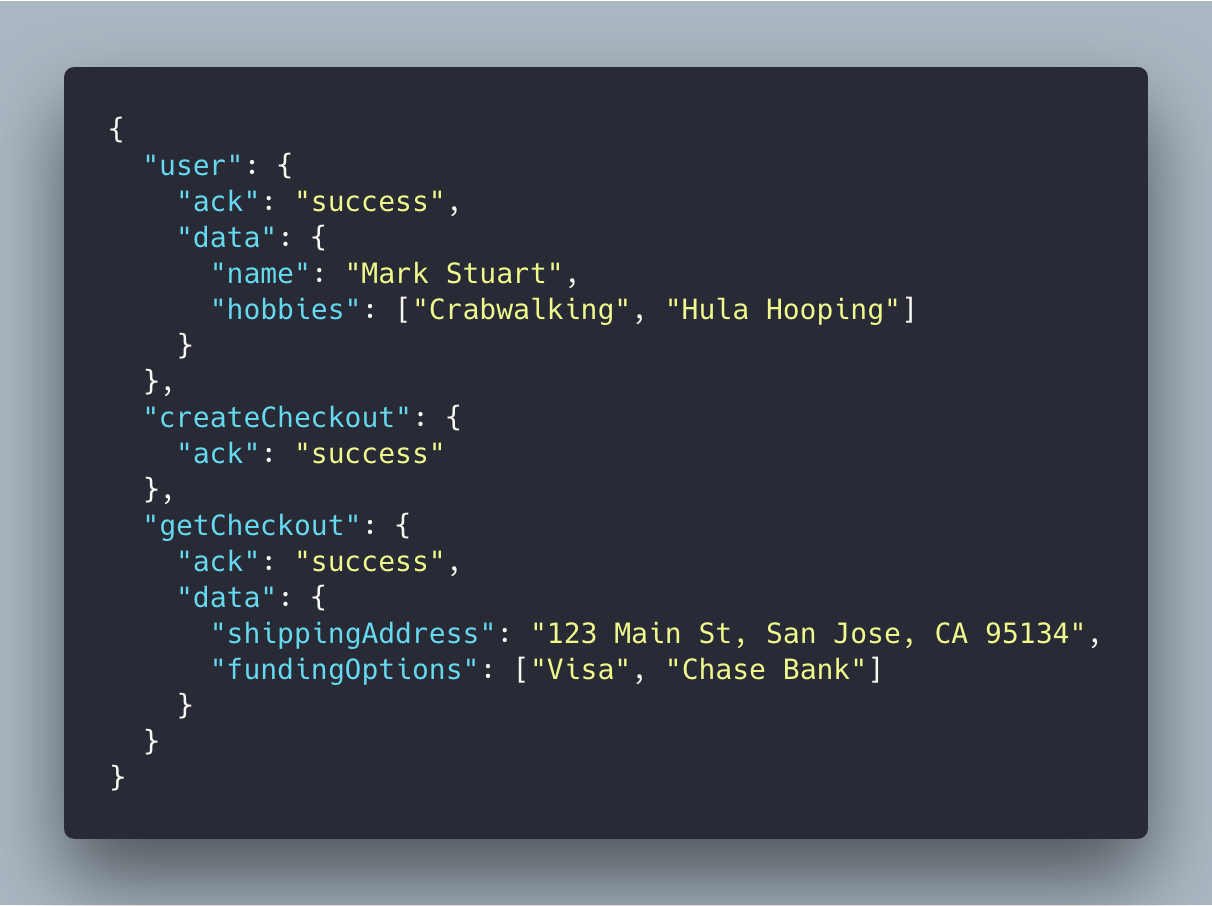
But, it was rarely used.
With Batch, we liked that clients control the size and shape of data, not servers. That freed us from having to tweak an orchestration API every time a client’s requirements changed. We didn’t like that clients had to intimately know how the underlying APIs worked. The request structure was painful enough for developers to not use it. We also wanted to be able to fetch specific fields, not large resources or objects.
Many companies (such as Facebook and Google) that have a Batch offering have the same problem. It’s treated as an optimized, but undocumented feature, not the first-class way to fetch data.
Batch REST was a step in the right direction, but wasn’t a game changer.