Handling 1 Million Requests per Minute with Golang

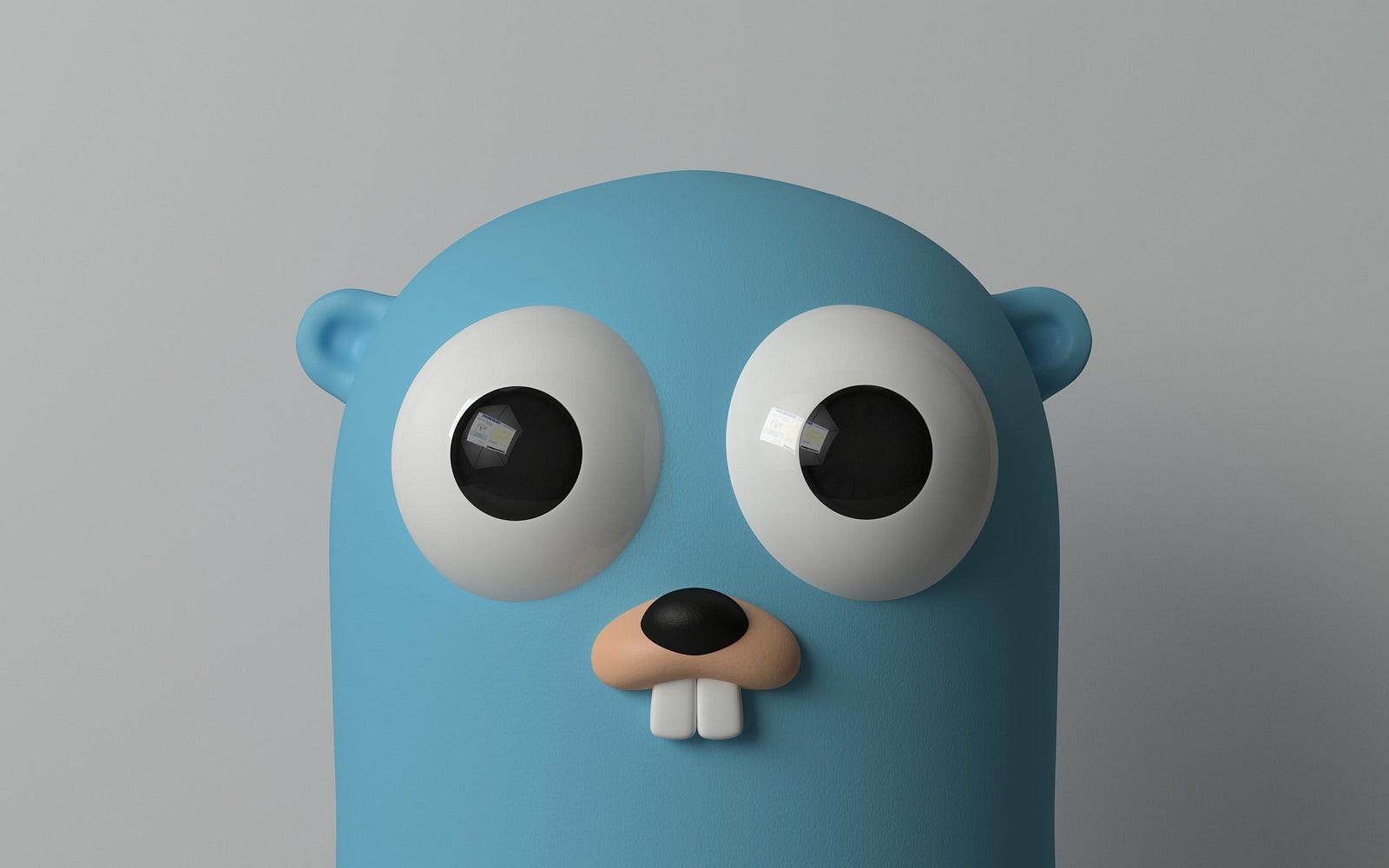
Handling 1 Million Requests per Minute with Golang
I have been working in the anti-spam, anti-virus and anti-malware industry for over 15 years at a few different companies, and now I know how complex these systems could end up being due to the massive amount of data we handle daily.
Currently I am CEO of
What is interesting is that for the last 10 years or so as a Software Engineer, all the web backend development that I have been involved in has been mostly done in Ruby on Rails. Don’t take me wrong, I love Ruby on Rails and I believe it’s an amazing environment, but after a while you start thinking and designing systems in the ruby way, and you forget how efficient and simple your software architecture could have been if you could leverage multi-threading, parallelization, fast executions and small memory overhead. For many years, I was a C/C++, Delphi and C# developer, and I just started realizing how less complex things could be with the right tool for the job.
I am not very big on the language and framework wars that the interwebs are always fighting about. I believe efficiency, productivity and code maintainability relies mostly on how simple you can architect your solution.
The Problem
While working on a piece of our anonymous telemetry and analytics system, our goal was to be able to handle a large amount of POST requests from millions of endpoints. The web handler would receive a JSON document that may contain a collection of many payloads that needed to be written to Amazon S3, in order for our map-reduce systems to later operate on this data.
Traditionally we would look into creating a worker-tier architecture, utilizing things such as:
- Sidekiq
- Resque
- DelayedJob
- Elasticbeanstalk Worker Tier
- RabbitMQ
- and so on…
And setup 2 different clusters, one for the web front-end and another for the workers, so we can scale up the amount of background work we can handle.
But since the beginning, our team knew that we should do this in Go because during the discussion phases we saw this could be potentially a very large traffic system. I have been using Go for about 2 years or so, and we had developed a few systems here at work but none that would get this amount of load.
We started by creating a few structures to define the web request payload that we would be receiving through the POST calls, and a method to upload it into our S3 bucket.
Naive approach to Go routines
Initially we took a very naive implementation of the POST handler, just trying to parallelize the job processing into a simple goroutine:
For moderate loads, this could work for the majority of people, but this quickly proved to not work very well at a large scale. We were expecting a lot of requests but not in the order of magnitude we started seeing when we deployed the first version to production. We completely understimated the amount of traffic.
The approach above is bad in several different ways. There is no way to control how many go routines we are spawning. And since we were getting 1 million POST requests per minute of course this code crashed and burned very quickly.
Trying again
We needed to find a different way. Since the beginning we started discussing how we needed to keep the lifetime of the request handler very short and spawn processing in the background. Of course, this is what you must do in the Ruby on Rails world, otherwise you will block all the available worker web processors, whether you are using puma, unicorn, passenger (Let’s not get into the JRuby discussion please). Then we would have needed to leverage common solutions to do this, such as Resque, Sidekiq, SQS, etc. The list goes on since there are many ways of achieving this.
So the second iteration was to create a buffered channel where we could queue up some jobs and upload them to S3, and since we could control the maximum number of items in our queue and we had plenty of RAM to queue up jobs in memory, we thought it would be okay to just buffer jobs in the channel queue.
And then to actually dequeue jobs and process them, we were using something similar to this:
To be honest, I have no idea what we were thinking. This must have been a late night full of Red-Bulls. This approach didn’t buy us anything, we have traded flawed concurrency with a buffered queue that was simply postponing the problem. Our synchronous processor was only uploading one payload at a time to S3, and since the rate of incoming requests were much larger than the ability of the single processor to upload to S3, our buffered channel was quickly reaching its limit and blocking the request handler ability to queue more items.
We were simply avoiding the problem and started a count-down to the death of our system eventually. Our latency rates kept increasing in a constant rate minutes after we deployed this flawed version.


The Better Solution
We have decided to utilize a common pattern when using Go channels, in order to create a 2-tier channel system, one for queuing jobs and another to control how many workers operate on the JobQueue concurrently.
The idea was to parallelize the uploads to S3 to a somewhat sustainable rate, one that would not cripple the machine nor start generating connections errors from S3. So we have opted for creating a Job/Worker pattern. For those that are familiar with Java, C#, etc, think about this as the Golang way of implementing a Worker Thread-Pool utilizing channels instead.
We have modified our Web request handler to create an instance of Job
struct with the payload and send into the JobQueue
channel for the workers to pickup.
During our web server initialization we create a Dispatcher
and call Run()
to create the pool of workers and to start listening for jobs that would appear in the JobQueue
.
dispatcher := NewDispatcher(MaxWorker)
dispatcher.Run()
Below is the code for our dispatcher implementation:
Note that we provide the number of maximum workers to be instantiated and be added to our pool of workers. Since we have utilized Amazon Elasticbeanstalk for this project with a dockerized Go environment, and we always try to follow the 12-factor methodology to configure our systems in production, we read these values from environment variables. That way we could control how many workers and the maximum size of the Job Queue, so we can quickly tweak these values without requiring re-deployment of the cluster.
var (
MaxWorker = os.Getenv("MAX_WORKERS")
MaxQueue = os.Getenv("MAX_QUEUE")
)
Immediately after we have deployed it we saw all of our latency rates drop to insignificant numbers and our ability to handle requests surged drastically.

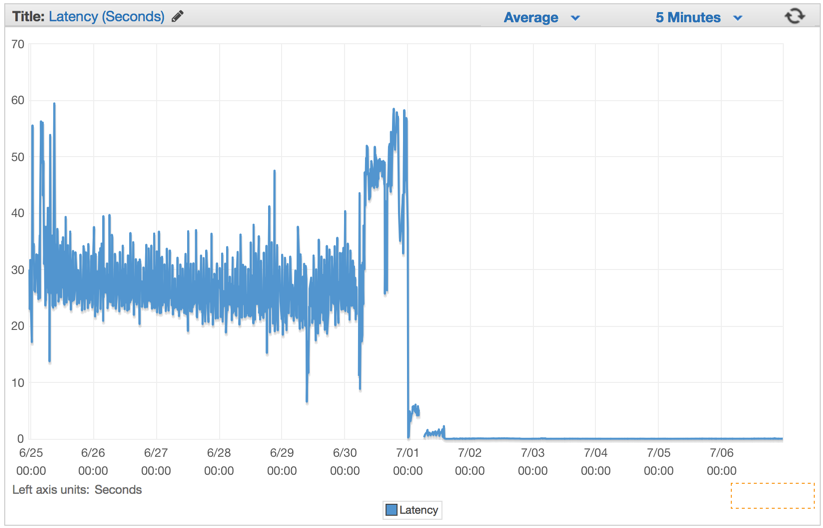
Minutes after our Elastic Load Balancers were fully warmed up, we saw our ElasticBeanstalk application serving close to 1 million requests per minute. We usually have a few hours during the morning hours in which our traffic spikes over to more than a million per minute.
As soon as we have deployed the new code, the number of servers dropped considerably from 100 servers to about 20 servers.

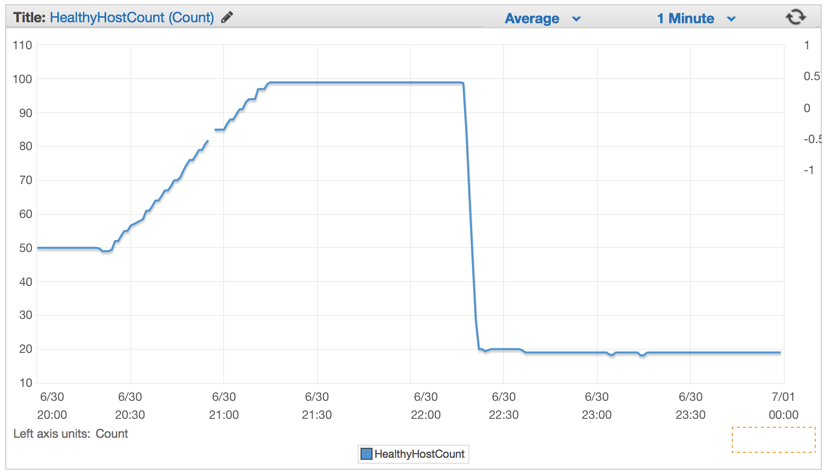
After we had properly configured our cluster and the auto-scaling settings, we were able to lower it even more to only 4x EC2 c4.Large instances and the Elastic Auto-Scaling set to spawn a new instance if CPU goes above 90% for 5 minutes straight.


Conclusion
Simplicity always wins in my book. We could have designed a complex system with many queues, background workers, complex deployments, but instead we decided to leverage the power of Elasticbeanstalk auto-scaling and the efficiency and simple approach to concurrency that Golang provides us out of the box.
It’s not everyday that you have a cluster of only 4 machines, that are probably much less powerful than my current MacBook Pro, handling POST requests writing to an Amazon S3 bucket 1 million times every minute.
There is always the right tool for the job. For sometimes when your Ruby on Rails system needs a very powerful web handler, think a little outside of the ruby eco-system for simpler yet more powerful alternative solutions.
相關推薦
Handling 1 Million Requests per Minute with Golang
Handling 1 Million Requests per Minute with GolangI have been working in the anti-spam, anti-virus and anti-malware industry for over 15 years at a few dif
Cross shard transactions at 10 million requests per second
Dropbox stores petabytes of metadata to support user-facing features and to power our production infrastructure. The primary system we use to store this
We're Celebrating 1 Million Page Views per Month!
They say people come to Python for the language and stay for the community. We couldn’t agree more! You mean the world to us, and we are honored to have
idea出現:error:java: Target level '1.7' is incompatible with source level '1.8'.解決辦法
tin get 技術 strong mark 更改 ID HR ati 當我們開始使用idea的時候,編譯jsp程序我們有可能出現編譯錯誤,然而我們的代碼又沒有什麽問題。 解決方法一:我們開始的時候可以通過修改java compiler來解決這樣的問題,點擊file菜
[轉]Magento 2 and 1 Million Products
course rman AS products strong desc 圖片 benchmark extension 本文轉自:https://www.goivvy.com/blog/magento-2-1-million-products Can Magento 2 h
Kafka to ClickHouse with Golang Driver
Kafka to ClickHouse with Golang Driver 前言 Base on Teleport 1.0 在之前的文章中我們提到過如果通過Hangout將Kafka中的資料接入ClickHouse中,相關文章。Hangout固然是一個很
Using encrypted private keys with Golang HTTPS server
Using encrypted private keys with Golang HTTPS serverYes, this is an Owl, protecting my trafficComing from a Java world (I am embarrassed enough), this hit
How listening to our 4.1 million users inspired a new product idea
How listening to our 4.1 million users inspired a new product ideaOriginally published on JOTFORM.COMYears ago, I was waiting in line at a paddle sports sh
Huawei aims to help train 1 million AI talents in 3 years
Technology giant Huawei aims to help train one million artificial intelligence (AI) talents in the next three years to boost the fast-expanding sector. Hua
Dating Site Owner to Repay $1 Million to Customers
The company that owns Christian Mingle, Jdate and other online dating sites will pay a $500,000 fine and nearly $1 million in restitution to customers whos
Buyer fragmentation matters: $1bn marketplaces have at least 1 million buyers
Buyer fragmentation matters: $1bn marketplaces have at least 1 million buyersThis is the third in a series of essays on my learnings and findings from a si
Mocking with Golang
Overview Writing unit tests are vital and ensures the integrity of your code. But sometimes it is hard to write tests for code that references external
Create a Slack bot with golang GopherAcademy
Create a Slack bot with golang Introduction In this post we’ll look at how to set up a quick Slack bot that receives m
Creating A Simple Web Server With Golang
Last Updated - 3rd December, 2018 Web servers are always a really cool and relatively simple project to get up and running when trying to learn
Parsing XML Files With Golang
In this tutorial we look at how you can effectively read in an XML file from the file system and then parse this file using Go’s “encoding/xml” Pac
Creating a RESTful API With Golang
In this article, I’m going to be showing you how we can create a simple Golang based REST API that serves up a JSON based response whenever it is h
Concurrency With Golang Goroutines
Welcome all! In this tutorial, we are going to be looking at how you can use goroutines within you Go based programs and subsequently improve the p
Parsing JSON files With Golang
Welcome all, in this tutorial, we are going to be taking a look at how you can read in JSON files, or JSON HTTP responses and parse them to your he
Executing System Commands With Golang
This tutorial is just a quick and simple tutorial as to how to execute system commands using Google’s GoLang programming language. Official Docume
How to Get to 1 Million Users for your Chatbot
How to Get to 1 Million Users for your ChatbotBased on Personal Experience & Working with the Top Chatbot CompaniesOver the past few months, I have wor