Prevalence-Induced Behavior and AI Self-Driving Cars
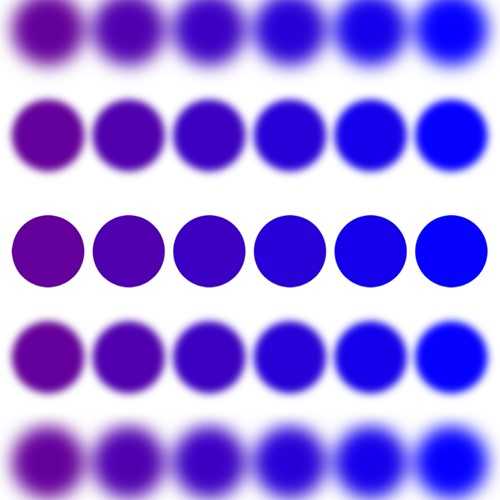
By Lance Eliot, the AI Trends Insider
During my daily commute on the hectic freeways of Southern California (SoCal), there are drivers that seem to believe that if they aggressively tailgate the car ahead of them (dangerously so!), such an effort will somehow make the traffic go faster. I am sure that these hostile drivers would gladly push or shove the car ahead of them as though they were at a child’s bumper cars ride at an amusement park, if they could do so legally.
I’m not quite convinced that their riding on the tail of the car ahead of them is really achieving what they hope for. Yes, there are some drivers that upon noticing they are being tailgated will speed-up, but a casual observation suggests it is not as many as perhaps the belligerent drivers assume will do so. There are even some drivers that once they spot a tailgater will actually pump their brakes lightly and tend to slow down, apparently believing that this will warn the other nosy driver to back-away and not be so pushy.
I’d guess that whenever the pushy tailgater gets the “I’ll stop you” driver ahead of them, it only makes the pushy driver want to go even faster and get more irked about the traffic. There have indeed been road rage incidents involving a “faster” driver that was upset about a “slower” driver and they decided to pull over to the side of the freeway and go to fisticuffs over the matter. Those kinds of wild west duke-it-out moments are a sight to behold and though it is not to be condoned it does make for some visual entertainment when otherwise stuck in snarled traffic.
Another concern about the pushy driver is that if the car ahead of them seems to be blocking progress, the pushiness spills over into other perilous maneuvers too. I’ve watched many times as a pushy driver came up to the bumper of another car, seemingly got frustrated that the car ahead wasn’t moving faster and decided to then scramble to pass the car by frantically swinging into another lane. I purposely herein use the word “frantically” because the pushy driver often does not look to see if there is any gap or room to make the lane change, and just does the lane change without a care as to how it might impact other drivers.
To make matters worse, when you have two pushy drivers that happen to end-up in the same place in traffic, they are then both wanting to be pushy in their own respective ways. Imagine you have plumbing at home that has a bit of a stoppage in it, and there is this gush of water that comes up to try and get around the stoppage. That’s what happens when two or more of the pushy drivers come upon a situation wherein there are other cars lolling along that seem to not be going as fast as the pushy drivers wish.
Once the two or more pushy drivers detect that another of their own species is nearby, they will frequently opt to turn the whole situation into a breast-beating gorilla-like challenge. One pushy driver will try to outdo another pushy driver. This turns any frantic maneuvers into even more alarming acts as they will veer towards other cars and use any tactic to get ahead of the other pushy car. I’ve seen such crazed drivers opt to use the emergency lane, illegally, in order to pass the other pushy driver, or swing into the HOV lane, illegally, and otherwise create accident-waiting moments on the freeway.
After my many years of witnessing this kind of aggressive driving behavior, you might assume that I’ve grown used to it and take it for granted. Though I don’t get overly alarmed about these pushy drivers, I still nonetheless have kept my driving edge in terms of detecting them and trying to stay clear of them. In other words, I don’t just ignore them and pretend they don’t exist, but instead I know about their antics and keep my wits about me to be wary of them. This happens somewhat subliminally, and I don’t seem to consciously be thinking about their ploys and have assimilated into my driving style the fact that they exist and how to contend with them.
During the Thanksgiving vacation break, I went up to a Northern California town that is relatively laid back in terms of how people drive and they weren’t nearly as aggressive as the usual Los Angeles drivers that I encounter on SoCal freeways and even on side streets such as in downtown Los Angeles. It was not immediately apparent to me that the driving style in this northern town was any different from my “norm” of driving in Southern California. It was only after having been in the town for a few days that I began to realize the difference.
There was an interesting phenomenon that overcame me during the first day or two of driving in this rather quiet town.
When I observed a car approaching me, doing so via my rear-view mirror, I would instinctively start to react as though the car was going to be a pushy driver. I did this repeatedly. Yet, by-and-large, the upcoming driver did not try the crazed pushiness dance that I was used to in Los Angeles. I wasn’t even aware that I was reacting until a passenger in my car noticed that I was slightly tensing up and moving forward when it wasn’t necessary to do so (I was trying to create a gap between me and the assumed pushy driver behind me, a form of defensive maneuver in reaction to a pushy driver).
What was happening to me?
Experiencing Prevalence-Induced Behavioral Change
I was likely experiencing prevalence-induced behavioral change. That’s a bit of jargon referring to one of the expanding areas of exploration about human judgment and social behaviors.
Something that is prevalence-induced refers to your having gotten used to having a lot of whatever the matter consists of (that’s the “prevalence” aspect), and you then assume that this high-frequency is still occurring even when it is no longer the case (that’s the “induced” aspect). You then even attempt to assert that the high-frequency is still there, though it no longer is (that’s the “behavioral change” aspect).
In my case, I was accustomed to the high-frequency of pushy drivers and so I overlaid that same perspective upon drivers in the small town and yet they weren’t being pushy drivers at all. My mental model was so ingrained to be watching for and reacting to pushy drivers that I saw pushy drivers when they weren’t any longer there.
I was fooling myself into believing there were pushy drivers, partially because I knew or expected that there must be pushy drivers wherever I am driving. Don’t misunderstand this point and assume that I was consciously calculating this aspect per se. I was not particularly aware that I was treating other drivers as though they were pushy drivers, until my passenger jogged me out of my mental fog and made me realize how I was driving. I was driving in the same manner I always drive. The problem though was that the driving situation had changed, but I had not changed my mental model.
There’s an old classic line that if you have a hammer the rest of the world looks like a nail. Since I had a mental model of being on alert for pushy drivers, I ascribed pushiness to drivers that didn’t deserve it. Let’s say that in Los Angeles there were 30% of drivers that were pushy, while in this town it was more like 3%. I was still expecting that 30% of the drivers would be pushy, and I mentally fulfilled this notion by assigning pushiness to their efforts, getting the proportion closer to my imagined 30%, regardless of whether their efforts actually met the traditional definition of being a pushy driver.
A study done by researchers at Harvard University, the University of Virginia, Dartmouth University, and NYU recently unveiled some fascinating experiments involving prevalence-induced behavioral change. Most notable perhaps was their study of blue dots and purple dots. The aspects about the dots seemed to catch the attention of the widespread media and was covered extensively both nationally and internationally.
In brief, they showed human subjects an array of 1,000 colored dots. The colors of the dots varied on a continuum of being very purple to being very blue. Subjects were to identify which dots were purple and which were blue (actually, they were asked to indicate which dots were blue and which were not blue). Let’s assume that the coverage of blue and purple dots was around half and half. After numerous trials of this activity, the researchers then decreased the number of blue dots and increased the number of purple dots (keeping the same total number of dots).
Some participants in the study got the now re-proportioned mixture of blue and purple dots (the experimental “treatment” group), while the control group participants got the same half-and-half mixture (these were the “stable prevalence” participants). The control group still identified the same overall proportion as before, which is handy because it suggests they were still performing as they had all along.
Meanwhile, the treatment group began to identify dots as blue that were now purple, doing so roughly to seemingly have the same balance of blue and purple dots as they had already gotten used to. One would not expect that they would do so. You would assume that if they were “objectively” examining the dots, they would have identified correctly the newer proportion and have done so by simply accurately stating which dots were blue and which were purple.
The experimenters decided to explore this further and did additional studies. They tried another version in which the number of blue dots was increased, and the number of purple dots was decreased, thus the opposite approach of the earlier experiment. What happened? Once again, the treatment group tended to overinflate by identifying the more prevalent blue dots as purple dots, arriving at the mixture level of the initial trials. The researchers did other variants of the same study including warning the subjects, but the result still came out roughly the same.
Just in case some might argue that dots are not much of a visually complex matter, they redid the experiment with 800 computer-generated human faces. The faces were varied on a continuum of appearing to be very threatening to being not very threatening. Experiments were done similarly to the dot’s procedures. Once again, the subjects tended to showcase that they would be influenced by the prevalence aspects.
Why does this matter? The prevalence-induced behavioral change can lead to problematic human behavior. In my story about driving in the small town, my reacting to non-existent pushiness could have inadvertently led to traffic accidents. I enlarged my notion of pushy drivers to include drivers that were not at all being pushy. I was assigning the color blue to purple dots.
Suppose a radiologist that is used to seeing MRI images that are over-and-over are ones with cancer, and then the radiologist comes upon an image that does not have cancer. The radiologist might ascribe cancer when it is not actually present, due to the prevalence-induced aspects. Not a good thing.
There is a danger that we as humans might act or make decisions that are based not on what is right there in front of us, but instead based on what our mental models lead us to believe is there. This might seem startling because you would assume that the facts are the facts, the blue dots are the blue dots and the purple dots are the purple dots. Of course, human interpretation and human foibles can differently interpret what we see, hear, taste, etc.
What does this have to do with AI self-driving cars?
At the Cybernetic AI Self-Driving Car Institute, we are developing AI software for self-driving cars. One aspect of AI self-driving cars is whether they will be able to do a better job at driving than humans can, and also whether the Machine Learning (ML) aspects of the AI will be subject to traditionally human-based foibles.
Allow me to elaborate.
I’d like to first clarify and introduce the notion that there are varying levels of AI self-driving cars. The topmost level is considered Level 5. A Level 5 self-driving car is one that is being driven by the AI and there is no human driver involved. For the design of Level 5 self-driving cars, the auto makers are even removing the gas pedal, brake pedal, and steering wheel, since those are contraptions used by human drivers. The Level 5 self-driving car is not being driven by a human and nor is there an expectation that a human driver will be present in the self-driving car. It’s all on the shoulders of the AI to drive the car.
For self-driving cars less than a Level 5, there must be a human driver present in the car. The human driver is currently considered the responsible party for the acts of the car. The AI and the human driver are co-sharing the driving task. In spite of this co-sharing, the human is supposed to remain fully immersed into the driving task and be ready at all times to perform the driving task. I’ve repeatedly warned about the dangers of this co-sharing arrangement and predicted it will produce many untoward results.
Let’s focus herein on the true Level 5 self-driving car. Much of the comments apply to the less than Level 5 self-driving cars too, but the fully autonomous AI self-driving car will receive the most attention in this discussion.
Here’s the usual steps involved in the AI driving task:
- Sensor data collection and interpretation
- Sensor fusion
- Virtual world model updating
- AI action planning
- Car controls command issuance
Another key aspect of AI self-driving cars is that they will be driving on our roadways in the midst of human driven cars too. There are some pundits of AI self-driving cars that continually refer to a Utopian world in which there are only AI self-driving cars on the public roads. Currently there are about 250+ million conventional cars in the United States alone, and those cars are not going to magically disappear or become true Level 5 AI self-driving cars overnight.
Indeed, the use of human driven cars will last for many years, likely many decades, and the advent of AI self-driving cars will occur while there are still human driven cars on the roads. This is a crucial point since this means that the AI of self-driving cars needs to be able to contend with not just other AI self-driving cars, but also contend with human driven cars. It is easy to envision a simplistic and rather unrealistic world in which all AI self-driving cars are politely interacting with each other and being civil about roadway interactions. That’s not what is going to be happening for the foreseeable future. AI self-driving cars and human driven cars will need to be able to cope with each other.
Pre-Trained Machine Learning System Might Not Know a Different Place
Returning to the topic of prevalence-induced behavioral change, let’s consider how this could come to play in the case of AI self-driving cars.
We’ll start by mulling over the nature of Machine Learning (ML) and AI self-driving cars. Machine Learning and especially deep learning using large-scale artificial neural networks are being used to be able to aid the processing of data that is collected by sensors on an AI self-driving car. When an image is captured via cameras on the AI self-driving car, the image is potentially processed by pumping it through a trained neural network.
A neural network might have been trained on the detection of street signs. Via analyzing the data of a street scene, the neural network can possibly determine that a Stop sign is up ahead or ascertain the posted speed limit based on a speed limit sign. Likewise, the neural network or an allied neural network might be have been trained on detecting the presence of pedestrians. By analyzing the street scene image, the neural network could be looking for the shape of the arms, legs, body and other physical facets that suggest a pedestrian is up ahead.
It is generally likely that for the time being these Machine Learning algorithms will be pre-trained and not be unleashed to try and adjust and learn new elements while in-the-field. Besides the tremendous amount of potential computing power that would be needed to learn on-the-fly, there would also be the potential danger that the “learning” might go off-kilter and not learn what we would want the system to learn.
For example, suppose the ML began to mistake fire hydrants as being pedestrians, or, perhaps worse so, it began interpreting pedestrians as being fire hydrants. Without some kind of more formalized checks-and-balances approach, allowing an on-the-fly machine learner on a standalone basis in the context of an AI self-driving car is dicey. More likely would be the collecting of data from AI self-driving cars up into the cloud of the auto maker or tech firm involved in the self-driving car, doing so via OTA (Over-The-Air) electronic communications, and the auto maker or tech firm would then use the data for adding machine learning (doing so across the entire fleet of AI self-driving cars). An updated neural network based on the added machine learning could then be pushed back down into the AI self-driving car via the OTA for use in execution of doing improved image analyses.
Besides trying to analyze aspects such as street signs and the presence of pedestrians, another potential use of the Machine Learning would be to look for patterns in traffic situations.
The better that the AI can be at detecting recognizable and relatively repeatable kinds of traffic patterns, the more that the AI can then be prepared to readily and rapidly deal with those particular idiosyncratic traffic aspects. If the AI is not versed in traffic patterns, it must try in real-time to cope with how to best act or react. Instead, if the traffic pattern is one that has been previously experienced and codified, along with having identified fruitful means of dealing with the traffic pattern, the AI can more gingerly drive the car and drive in a human-like defensive manner.
Suppose an AI self-driving car is driving in Los Angeles and taking me to my office each day. Over and over it collects traffic data indicative of aggressive drivers, which are aplenty here (you can spot them like fish in a barrel). The data gets uploaded to the cloud. An analysis is undertaken and the deep learning adjusts the neural networks, which are then reloaded into my AI self-driving car. Gradually, over weeks or months, the AI self-driving car gets better and better at contending with the pushy drivers.
Here’s my question for you – do you think it is possible that the AI might eventually reach a point of anticipating and expecting pushy drivers, so much that it then if taken to a new locale might ascribe the pushiness to non-pushy drivers?
In essence, I am suggesting that the prevalence-induced behavioral change that I had personally experienced, as a human being (which, I declare to you that I am indeed a human being – please rest assured that I am not a robot!), could very well happen to the AI of an AI self-driving car. It is reasonable and conceivable that upon going to that little town up in Northern California, the AI might be watching for aggressive drivers and assume that drivers that aren’t pushy are actually indeed pushy, based on the prevalence-induced aspects.
The AI might see blue dots where there are purple dots, if you get my drift.
There are a myriad of other ways in which the prevalence-induced behavioral aspects can arise in the AI of a self-driving car. It will be crucial for AI developers to realize that this kind of human judgement “impairment” can also strike at the underpinnings of the Machine Learning and artificial neural networks used in AI (I’ll add that this is true beyond just the topic of self-driving cars, and thus it is something to be considered for any kind of AI system).
How to catch this phenomenon will be a key programming concern for any savvy AI developer that does not want their AI to fall into this prevalence trap.
Besides the AI developers themselves detecting it, which is a human manual kind of check-and-balance, a truly self-aware AI system should have internal system mechanisms to be on the look for this judgement malady.
The AI’s self-awareness would be the last line of defense since it would be the portion that while the AI self-driving is in the midst of driving, it would be the prod that would nudge the AI to realize what is afoot. I had mentioned that the passenger in my car was my prod, though I wish it had been my own mind that had noticed it (probably was enjoying being on vacation too much!).
Given that we know that prevalence-induced biases can creep into data and thus into a ML-based system, we need to have some kind of automated anti-prevalence detection and antidote. During my morning commutes, I’ll be working on that solutions, along with continuing to keep a watchful eye on those pushy drivers.
Copyright 2018 Dr. Lance Eliot
This content is originally posted on AI Trends.