【深度學習】perceptron(感知機)
阿新 • • 發佈:2020-04-01
[TOC]
個人學習筆記,有興趣的朋友可參考。
### 1.感知機的描述
感知機(perceptron)由美國學者Frank Rosenblatt在1957年提出來的。是作為神經網路(深度學習)的起源的演算法、
學習感知機的構造也就是學習通向神經網路和深度學習的一種重要思想
感知機接收多個輸入訊號,輸出一個訊號。如下圖:
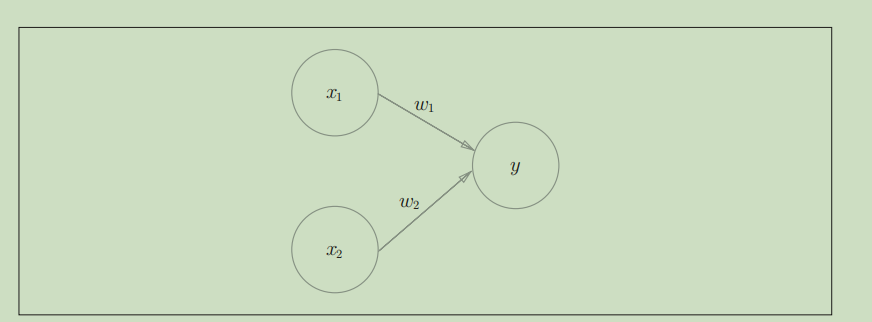
其中:
* x1、x2是輸入訊號,y是輸出訊號
* w1、w2是權重
* 圖中的○,代表一個個“神經元”
* 通過一個固定的權重演算法(w1x1、w2x2),當超過θ這個閾值,輸出1,代表“神經元被啟用”。否則為0,代表未啟用、
公式如下:
$$
Y=
\begin{cases}
0,(w1x1+w2x2<= θ)\\
1,(w1x1+w2x2> θ)
\end{cases}
$$
### 2.感知機解決簡單邏輯電路,與門的問題。
與門的真值表如下:
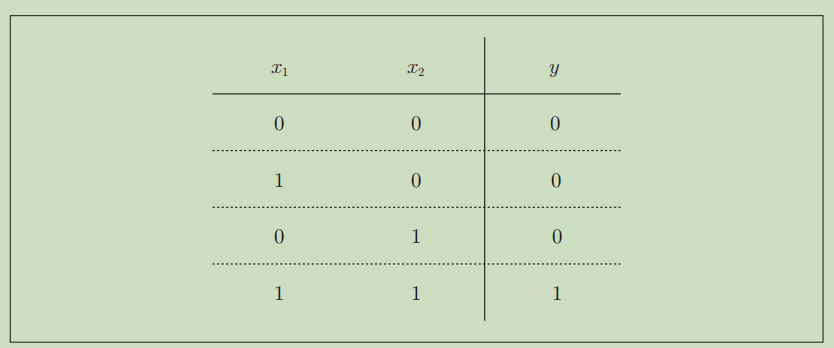
就是兩個輸入都為1的情況下,輸出才為1。
程式碼如下:
```python
def AND(x1,x2):
w1,w2,theta =0.5,0.5,0.6
tmp =x1*w1 +x2*w2
if tmp <= theta:
return 0
elif tmp >theta:
return 1
print(AND(0,0))
print(AND(1,0))
print(AND(0,1))
print(AND(1,1))
```
演示效果如下:
```
(zsdpy1) zsd@zsd-virtual-machine:~/ZAI$ python section04.py
0
0
0
1
```
### 2.多層感應機,解決異或門
異或門的真值表
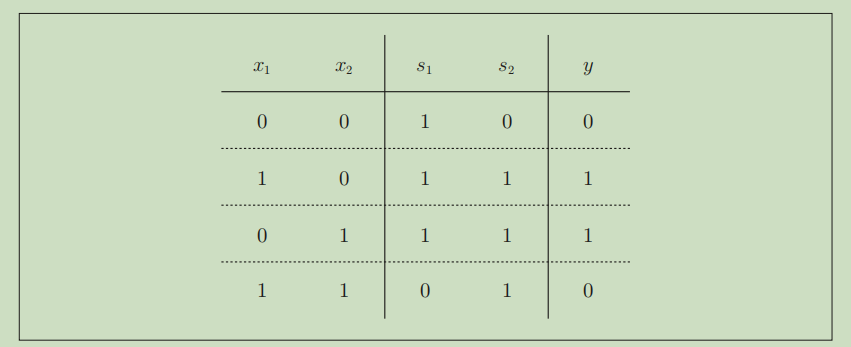
可以看到,異或門用一層沒有辦法解決。所以我們可以再來一個"疊加層"、通過多層的結構。來達到異或門的效果。
如下圖:
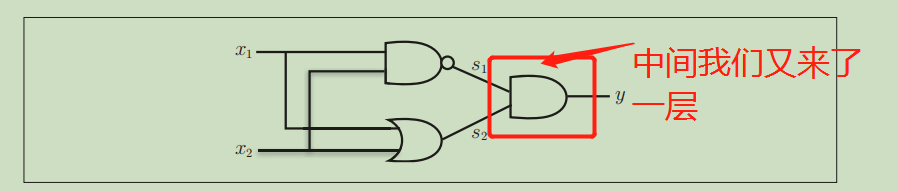
程式碼的實現,可能需要把`與門`,`非門`,`與非門`的函式都實現一遍。通過它們的組合完成`異或門`(大學時期學的邏輯電路終於派上了一點點用場了)
程式碼如下:
```python
import numpy as np
def AND(x1,x2):
w1,w2,theta =0.5,0.5,0.6
tmp =x1*w1 +x2*w2
if tmp <= theta:
return 0
elif tmp >theta:
return 1
def OR(x1, x2):
x = np.array([x1, x2])
w = np.array([0.5, 0.5])
b = -0.2
tmp = np.sum(w*x) + b
if tmp <= 0:
return 0
else:
return 1
def NAND(x1, x2):
x = np.array([x1, x2])
w = np.array([-0.5, -0.5])
b = 0.7
tmp = np.sum(w*x) + b
if tmp <= 0:
return 0
else:
return 1
def XOR(x1, x2):
s1 = NAND(x1, x2)
s2 = OR(x1, x2)
y = AND(s1, s2)
return y
if __name__ == '__main__':
for xs in [(0, 0), (1, 0), (0, 1), (1, 1)]:
y = XOR(xs[0], xs[1])
print(str(xs) + " -> " + str(y))
```
演示效果如下:
```
(zsdpy1) zsd@zsd-virtual-machine:~/ZAI$ python section05.py
(0, 0) -> 0
(1, 0) -> 1
(0, 1) -> 1
(1, 1) -